Remote sensing image binary change detection method based on feature deviation alignment
A remote sensing image and change detection technology, applied in the field of remote sensing image processing, can solve problems such as unaccounted for feature deviation, false detection, and dual-temporal image feature deviation
- Summary
- Abstract
- Description
- Claims
- Application Information
AI Technical Summary
Problems solved by technology
Method used
Image
Examples
Embodiment Construction
[0051] In order to make the technical method of the present invention clearer, the specific implementation of the present invention will be described in further detail below in conjunction with the accompanying drawings, but the specific examples described are only to illustrate the spirit of the present invention, and the implementation is not limited thereto.
[0052] Step 1. Construct a dual-temporal remote sensing image change detection dataset and perform preprocessing.
[0053] Specifically, step 1 further includes:
[0054] Step 1.1, the present invention selects the open source WHU Building building change detection data set of the network to construct a dual-temporal remote sensing image change detection data set, which includes two dual-temporal remote sensing images, which were taken in 2012 and 2016 respectively, and the image size is 15354×32057, with a resolution of 0.3 meters, covering an area of 20 square kilometers. Since the original image is a large-scale...
PUM
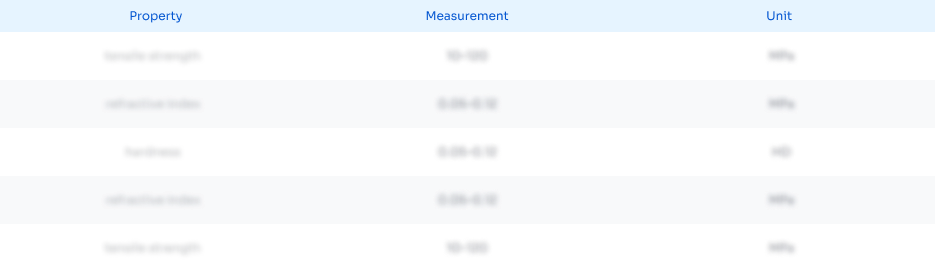
Abstract
Description
Claims
Application Information
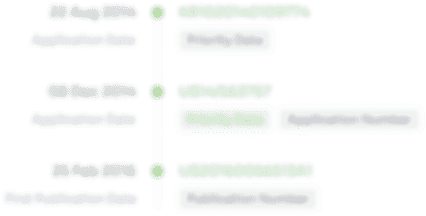
- Generate Ideas
- Intellectual Property
- Life Sciences
- Materials
- Tech Scout
- Unparalleled Data Quality
- Higher Quality Content
- 60% Fewer Hallucinations
Browse by: Latest US Patents, China's latest patents, Technical Efficacy Thesaurus, Application Domain, Technology Topic, Popular Technical Reports.
© 2025 PatSnap. All rights reserved.Legal|Privacy policy|Modern Slavery Act Transparency Statement|Sitemap|About US| Contact US: help@patsnap.com