Deep learning non-intrusive load monitoring method based on unsupervised optimization
A load monitoring, non-invasive technology, applied in the direction of neural learning methods, measuring electricity, measuring devices, etc., can solve problems such as poor self-learning ability, high cost, and lack of low-frequency NILM precision information, so as to save power expenditure and improve Accuracy, the effect of enhancing self-learning ability
- Summary
- Abstract
- Description
- Claims
- Application Information
AI Technical Summary
Problems solved by technology
Method used
Image
Examples
Embodiment
[0047] A deep learning non-intrusive load monitoring method based on unsupervised optimization, such as figure 1 As shown, the non-intrusive load monitoring method is realized by using the neural network neural learning optimized by unsupervised learning and PQ decoupling Farah. The non-invasive load monitoring method includes the following steps:
[0048] S1. Use the power monitoring equipment to extract the user's individual load and load cluster information;
[0049] Such as figure 2 , image 3 As shown, the voltage and current monitoring equipment is used to extract the power data, power factor and load cluster switching action of each individual load and load cluster of the user, and arrange them in time as the neural network training data. The neural network training input data includes the total active power of the load cluster Power, reactive power, switching action, time and date, and generate a power matrix from the measured data, ready to enter the next step of p...
PUM
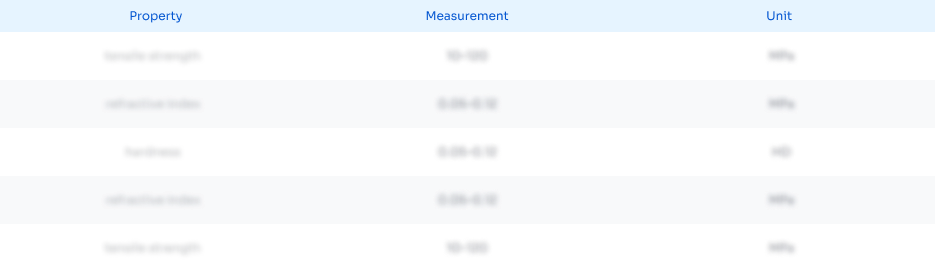
Abstract
Description
Claims
Application Information
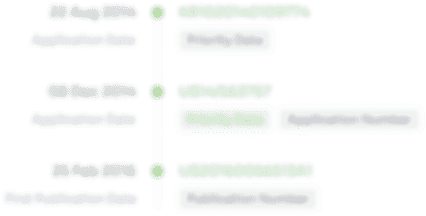
- R&D Engineer
- R&D Manager
- IP Professional
- Industry Leading Data Capabilities
- Powerful AI technology
- Patent DNA Extraction
Browse by: Latest US Patents, China's latest patents, Technical Efficacy Thesaurus, Application Domain, Technology Topic, Popular Technical Reports.
© 2024 PatSnap. All rights reserved.Legal|Privacy policy|Modern Slavery Act Transparency Statement|Sitemap|About US| Contact US: help@patsnap.com