Cross-domain image example level active labeling method
An example and image technology, applied in the fields of instruments, character and pattern recognition, calculation models, etc., can solve the problems of difficulty in improving model performance and difficulty in acquiring target domain data, and achieve the effect of low cost, reduced participation cost, and reduced difficulty.
- Summary
- Abstract
- Description
- Claims
- Application Information
AI Technical Summary
Problems solved by technology
Method used
Image
Examples
Embodiment Construction
[0041] The present invention will be further described below in conjunction with the accompanying drawings.
[0042] Such as figure 1 Shown is the working flow diagram of the digital image automatic labeling device. Assume that initially there is a small number of fully labeled training image data sets, including two parts from the source domain and the target domain; and a collected knowledge-rich unsupervised source domain sample pool The device first uses initial labeled data training to obtain a basic predictive model. Then, the model pairs the source domain data set The images are predicted, and the object detection prediction results of each unlabeled image and the domain classification results of images and examples are obtained. A utility score for each example is computed from the model output. After the candidate examples are post-processed, they are sorted according to the score, and the supervision information of the examples is queried from the user from hig...
PUM
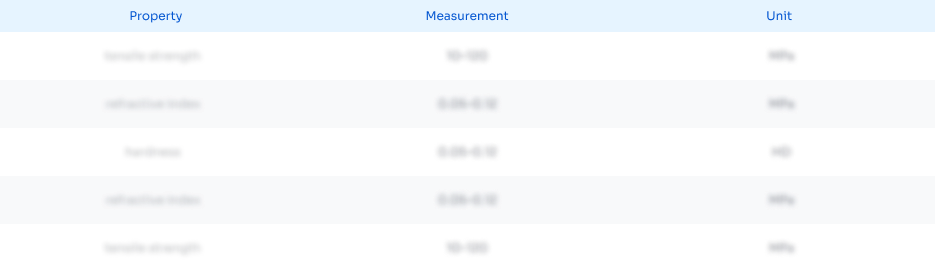
Abstract
Description
Claims
Application Information
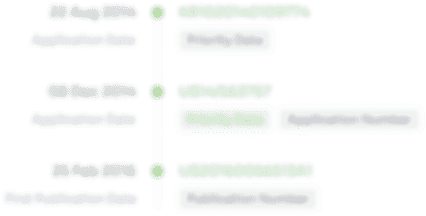
- R&D
- Intellectual Property
- Life Sciences
- Materials
- Tech Scout
- Unparalleled Data Quality
- Higher Quality Content
- 60% Fewer Hallucinations
Browse by: Latest US Patents, China's latest patents, Technical Efficacy Thesaurus, Application Domain, Technology Topic, Popular Technical Reports.
© 2025 PatSnap. All rights reserved.Legal|Privacy policy|Modern Slavery Act Transparency Statement|Sitemap|About US| Contact US: help@patsnap.com