Neural network model training method and device
A neural network model and training method technology, applied in the field of continuous learning scenarios, can solve problems such as inability to extract, inability to comprehensively extract features, and inability to obtain input data, and achieve the effect of improving classification accuracy
- Summary
- Abstract
- Description
- Claims
- Application Information
AI Technical Summary
Problems solved by technology
Method used
Image
Examples
Embodiment Construction
[0040] In order to make the objects, technical solutions, and advantages of the present disclosure more apparent, exemplary embodiments according to the present disclosure will be described in detail below with reference to the accompanying drawings. Apparently, the described embodiments are only some of the embodiments of the present disclosure, rather than all the embodiments of the present disclosure, and it should be understood that the present disclosure is not limited by the exemplary embodiments described here.
[0041] The solutions provided in the embodiments of the present application relate to neural network-based classification techniques in the field of artificial intelligence, and are specifically described through the following embodiments. It should be noted that although the following embodiments are described in the context of a classification task in an image recognition scene, the application scene of the present invention is not limited thereto, and may als...
PUM
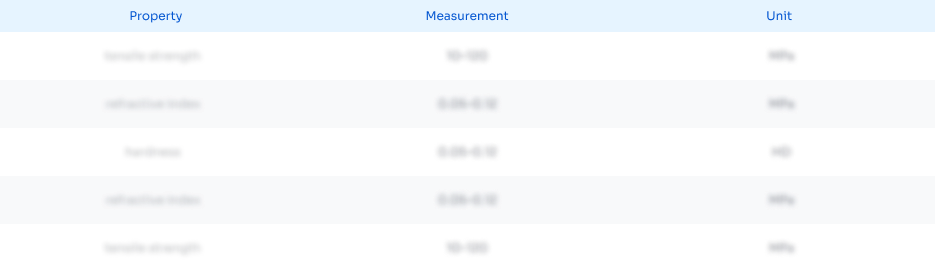
Abstract
Description
Claims
Application Information
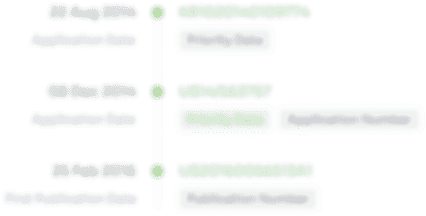
- R&D Engineer
- R&D Manager
- IP Professional
- Industry Leading Data Capabilities
- Powerful AI technology
- Patent DNA Extraction
Browse by: Latest US Patents, China's latest patents, Technical Efficacy Thesaurus, Application Domain, Technology Topic, Popular Technical Reports.
© 2024 PatSnap. All rights reserved.Legal|Privacy policy|Modern Slavery Act Transparency Statement|Sitemap|About US| Contact US: help@patsnap.com