Model detection method based on deep learning student online learning efficiency prediction
A model detection and deep learning technology, which is applied in the field of model detection for students' online learning efficiency prediction, can solve problems such as time lag, and achieve the effect of simple method, fast operation speed, and simplified construction steps
- Summary
- Abstract
- Description
- Claims
- Application Information
AI Technical Summary
Problems solved by technology
Method used
Image
Examples
Embodiment 1
[0033] Taking 1000 images from the image database as an example, the model detection method based on deep learning students' online learning efficiency prediction of this embodiment consists of the following steps (see figure 1 ):
[0034] (1) Obtain raw data and features
[0035] Take 1000 images from the image library and divide them into training set and test set, of which 700 are training sets and 300 are test sets. The convolutional neural network model is trained, and the test set is used for testing. After the test, the feature set and feature set are obtained. Add the state label as the Bayesian training set, put the Bayesian training set into the naive Bayesian model, obtain the state prediction model, intercept the user's use image, convert it into a picture set by frame, and use the convolutional neural network to obtain the user's image The eigenvector F, the eigenvector F is F 1 , F 2 , F 3 , F 4 , F 5 , F 6 A collection of vectors, that is, F ∈ {F 1 , F ...
Embodiment 2
[0058] Taking 1000 images from the image library as an example, the model detection method based on deep learning students' online learning efficiency prediction in this embodiment consists of the following steps:
[0059] (1) Obtain raw data and features
[0060] This step is the same as in Example 1.
[0061] (2) Determine the student's learning status
[0062] This step is the same as in Example 1.
[0063] (3) Determine the action state trajectory sequence
[0064] Connect the states at each moment by moment to obtain the state sequence of each action. The state sequence is:
[0065]
[0066] Among them, S∈{S 1 , S 2 ,...,S n} is the state, A∈{A 1 、A 2 ,...,A m} is an action, n and m are finite positive integers, the value of n in this embodiment is 32, and the value of m is 5, the described actions are: talking, yawning, not looking at the screen, closing eyes, sitting Not correct.
[0067] Other steps are the same as in Example 1.
[0068] Complete the mod...
Embodiment 3
[0070] Taking 1000 images from the image library as an example, the model detection method based on deep learning students' online learning efficiency prediction in this embodiment consists of the following steps:
[0071] (1) Obtain raw data and features
[0072] This step is the same as in Example 1.
[0073] (2) Determine the student's learning status
[0074] This step is the same as in Example 1.
[0075] (3) Determine the action state trajectory sequence
[0076] Connect the states at each moment by moment to obtain the state sequence of each action. The state sequence is:
[0077]
[0078] Among them, S∈{S 1 , S 2 ,...,S n} is the state, A∈{A 1 、A 2 ,...,A m} is an action, n and m are finite positive integers, the value of n in this embodiment is 64, and the value of m is 5, the described actions are: talking, yawning, not looking at the screen, closing eyes, sitting Not correct.
[0079] Other steps are the same as in Example 1.
[0080] Complete the mod...
PUM
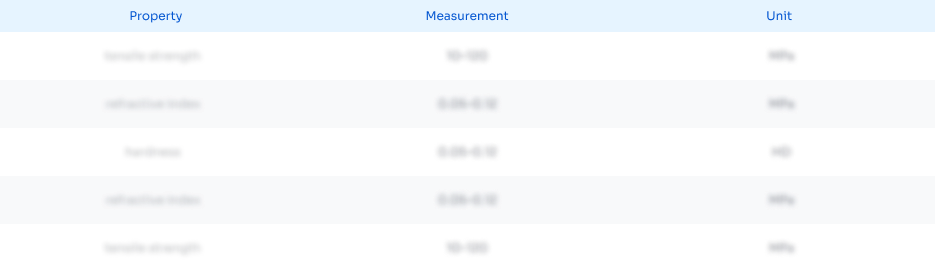
Abstract
Description
Claims
Application Information
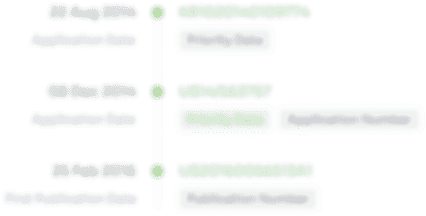
- R&D Engineer
- R&D Manager
- IP Professional
- Industry Leading Data Capabilities
- Powerful AI technology
- Patent DNA Extraction
Browse by: Latest US Patents, China's latest patents, Technical Efficacy Thesaurus, Application Domain, Technology Topic, Popular Technical Reports.
© 2024 PatSnap. All rights reserved.Legal|Privacy policy|Modern Slavery Act Transparency Statement|Sitemap|About US| Contact US: help@patsnap.com