Iterative hyperspectral image lossless compression method based on group low-rank representation
A technology of hyperspectral image, low rank representation, applied in image communication, instrument, character and pattern recognition, etc., can solve problems such as affecting compression results, inaccuracy, unstable k-means clustering results, etc., to enhance stability , the effect of high compression ratio
- Summary
- Abstract
- Description
- Claims
- Application Information
AI Technical Summary
Problems solved by technology
Method used
Image
Examples
Embodiment 1
[0026] After decades of development, the amount of data acquired by imaging spectrometers has expanded rapidly with the continuous improvement of spatial resolution and spectral resolution, and its huge amount of data has caused a huge burden on the storage and transmission of hyperspectral images. Seriously restricting the application prospects of hyperspectral images. Therefore, in order to improve storage and transmission efficiency and reduce costs, the present invention proposes an iterative hyperspectral image lossless compression method based on group low-rank representations.
[0027] The present invention is an iterative hyperspectral image lossless compression method based on group low-rank representation, see figure 1 , including the following steps:
[0028] (1) Define a spectral angle similarity measurement method: the spectral angle similarity measurement method of the present invention combines spectral angle and Euclidean distance to measure the similarity of ...
Embodiment 2
[0039] The iterative hyperspectral image lossless compression method based on group low-rank representation is the same as embodiment 1, the spectral angle similarity measurement method described in step (1), the spectral angle similarity measurement method combines spectral angle and Euclidean distance to hyperspectral image The similarity measure and its similarity measure formula are expressed as follows:
[0040]
[0041] in, Indicates the spectral line x and the spectral line The similarity of , the spectral line x is any spectral line participating in the similarity measurement, and the spectral line is another spectral line that needs to be compared with the spectral line x, Indicates the spectral line x and the spectral line The spectral angular distance between Indicates the spectral line x and the spectral line The spectral angular distance between, NA means spectral line x and spectral line The maximum spectral angular distance between, N L Indicat...
Embodiment 3
[0044] The iterative hyperspectral image lossless compression method based on the group low-rank representation is the same as embodiment 1-2, and the rough clustering initialization of the original image described in step (2) includes the following steps:
[0045] (2a) Select seed points: randomly select K seed points in the input hyperspectral image, and K is selected as 400 in this example.
[0046] (2b) Calculate the search range of rough clustering initialization seed points: input the expected number of superpixels K, assuming that the number of spectral lines of a hyperspectral image is N, then the search range calculation formula of a seed point is:
[0047]
[0048] L is the search step size, and the unit is the number of spectral lines. Since the calculation result of L is not necessarily an integer, the calculation result is an approximate value. In this example, N is 79099776 spectral lines, and the obtained L is 197749 spectral lines.
[0049] (2c) Assign a ro...
PUM
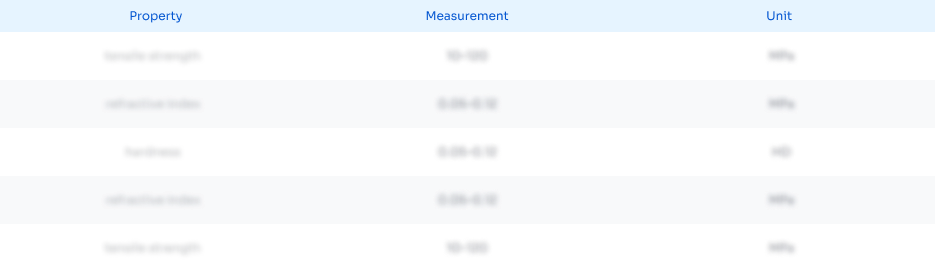
Abstract
Description
Claims
Application Information
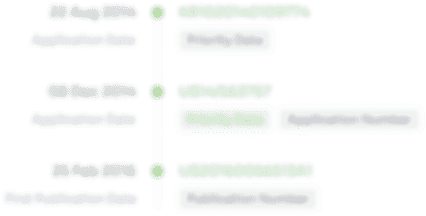
- R&D Engineer
- R&D Manager
- IP Professional
- Industry Leading Data Capabilities
- Powerful AI technology
- Patent DNA Extraction
Browse by: Latest US Patents, China's latest patents, Technical Efficacy Thesaurus, Application Domain, Technology Topic, Popular Technical Reports.
© 2024 PatSnap. All rights reserved.Legal|Privacy policy|Modern Slavery Act Transparency Statement|Sitemap|About US| Contact US: help@patsnap.com