TE process time sequence prediction method based on transfer entropy and long short-term memory network
A long-short-term memory and time series prediction technology, which is applied in neural learning methods, biological neural network models, complex mathematical operations, etc., can solve the problems of slow training rate and low accuracy of time series prediction, so as to speed up training speed and improve prediction accuracy Effect
- Summary
- Abstract
- Description
- Claims
- Application Information
AI Technical Summary
Problems solved by technology
Method used
Image
Examples
Embodiment Construction
[0053] The TE process is a model for simulating the actual process industrial system. According to the five parts contained in the process model, the Tennessee-Eastman process can be divided into five subunits, which are recorded as stirred reactor unit, condenser unit, Product separator unit, stripper unit and compressor unit. The present invention selects the stirred reactor unit to verify the validity of the method. Table 1 shows the 9 variables included in the stirred reactor unit of the TE process, and the reactor temperature is taken as the output variable of time series prediction.
[0054] Table 1 TE process stirred reactor unit variables
[0055] Table 1 Variables of TE process stirred reactor unit
[0056]
[0057] Based on the above description, according to the content of the invention, the specific process is implemented in spyder using the python language as follows:
[0058] step1: Take 958 sets of data under normal operation conditions, a total of 9 varia...
PUM
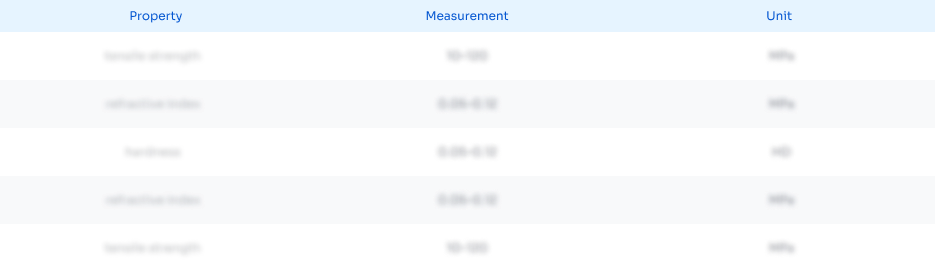
Abstract
Description
Claims
Application Information
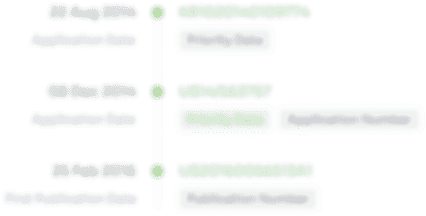
- R&D Engineer
- R&D Manager
- IP Professional
- Industry Leading Data Capabilities
- Powerful AI technology
- Patent DNA Extraction
Browse by: Latest US Patents, China's latest patents, Technical Efficacy Thesaurus, Application Domain, Technology Topic, Popular Technical Reports.
© 2024 PatSnap. All rights reserved.Legal|Privacy policy|Modern Slavery Act Transparency Statement|Sitemap|About US| Contact US: help@patsnap.com