Underwater vehicle target area floating control method based on double-commentator reinforcement learning technology
An underwater vehicle and target area technology, which is applied to neural learning methods, underwater ships, underwater operation equipment, etc., can solve the problem of the increase in the number of Q values, the slow convergence speed of algorithm training, easy acquisition without consideration, and reliable performance Expert data and other issues, to achieve good control effect, fast convergence effect
- Summary
- Abstract
- Description
- Claims
- Application Information
AI Technical Summary
Problems solved by technology
Method used
Image
Examples
Embodiment 1
[0098] A method for controlling the floating of an underwater vehicle target area based on double-critician reinforcement learning technology. The implementation process of the present invention is divided into two parts, the task environment construction stage and the floating strategy training stage, including the following steps:
[0099] 1. Define the task environment and model:
[0100] 1-1. Construct the task environment of the target area where the underwater vehicle is located and the dynamic model of the underwater vehicle;
[0101] Using the python language to write the underwater vehicle simulation environment task environment in the vscode integrated compilation environment, the geographic coordinate system E-ξηζ of the constructed simulated pool map is as follows image 3 As shown, the size of the three-dimensional pool is set to 50 meters * 50 meters * 50 meters, and the successful floating area of the target area is a cylindrical area with the center of the wa...
PUM
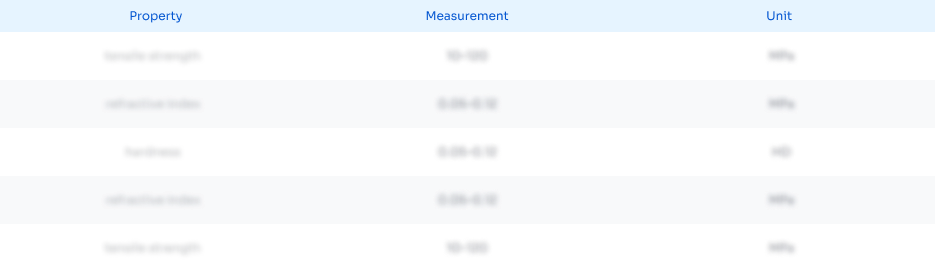
Abstract
Description
Claims
Application Information
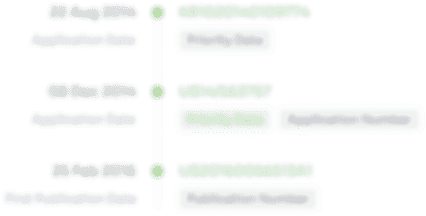
- R&D Engineer
- R&D Manager
- IP Professional
- Industry Leading Data Capabilities
- Powerful AI technology
- Patent DNA Extraction
Browse by: Latest US Patents, China's latest patents, Technical Efficacy Thesaurus, Application Domain, Technology Topic, Popular Technical Reports.
© 2024 PatSnap. All rights reserved.Legal|Privacy policy|Modern Slavery Act Transparency Statement|Sitemap|About US| Contact US: help@patsnap.com