A Guided Semantic Segmentation Method Based on Image Boundary Knowledge Transfer
A semantic segmentation and boundary technology, applied in the field of guided semantic segmentation based on image boundary knowledge transfer, can solve problems such as difficulty in specifying specific semantic segmentation and complex labeling samples.
- Summary
- Abstract
- Description
- Claims
- Application Information
AI Technical Summary
Problems solved by technology
Method used
Image
Examples
Embodiment Construction
[0039] Specific embodiments of the present invention will be described in detail below in conjunction with the accompanying drawings. It should be understood that the specific embodiments described here are only used to illustrate and explain the present invention, and are not intended to limit the present invention.
[0040] The present invention aims to solve the problem that the current semantic segmentation method based on deep learning requires a large number of annotations. When human beings perform visual perception, they do not need to know the category of the image to segment the semantic target well only by the edge of the object. However, the existing semantic segmentation methods are all based on the category labeling supervised segmentation network of the image to achieve the segmentation of the corresponding category target. . In order to solve the problem that the deep network needs a large number of annotations and specified semantic target segmentation, the p...
PUM
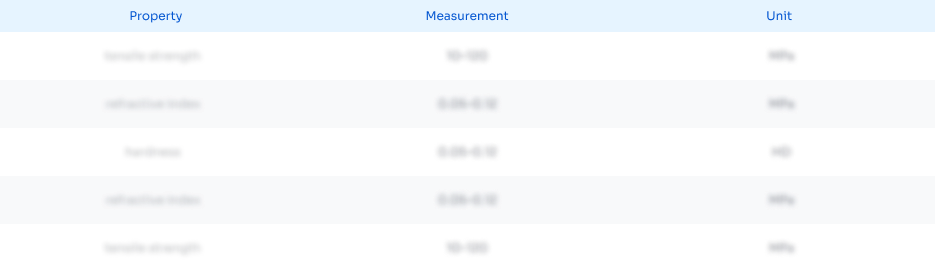
Abstract
Description
Claims
Application Information
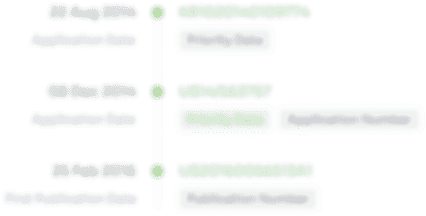
- Generate Ideas
- Intellectual Property
- Life Sciences
- Materials
- Tech Scout
- Unparalleled Data Quality
- Higher Quality Content
- 60% Fewer Hallucinations
Browse by: Latest US Patents, China's latest patents, Technical Efficacy Thesaurus, Application Domain, Technology Topic, Popular Technical Reports.
© 2025 PatSnap. All rights reserved.Legal|Privacy policy|Modern Slavery Act Transparency Statement|Sitemap|About US| Contact US: help@patsnap.com