Earth observation image semantic segmentation method based on self-supervised learning
An earth observation and semantic segmentation technology, applied in the fields of semantic segmentation and computer vision, can solve the problems of difficulty in extracting features and insufficient segmentation accuracy by semantic segmentation methods, achieve high semantic segmentation accuracy, and improve the effect of regional feature extraction capabilities.
- Summary
- Abstract
- Description
- Claims
- Application Information
AI Technical Summary
Problems solved by technology
Method used
Image
Examples
Embodiment Construction
[0046] The present invention will be further described below in conjunction with the accompanying drawings and embodiments, and the present invention includes but not limited to the following embodiments.
[0047] Such as figure 1 As shown, the present invention provides a method for semantic segmentation of earth observation images based on self-supervised learning, and its specific implementation process is as follows:
[0048] 1. Dataset preprocessing
[0049] Divide the earth observation image data set to be processed (such as the ISPRS Potsdam data set) into two parts, the training set and the test set, according to the ratio of 8:2. Among them, only a small number of images in the training set, that is, 10% of the images are labeled, and the rest of the images are not. with labels. After the images in the data set are cut, augmentation operations such as flip transformation, random rotation transformation, up, down, left, and right translation transformation, random cr...
PUM
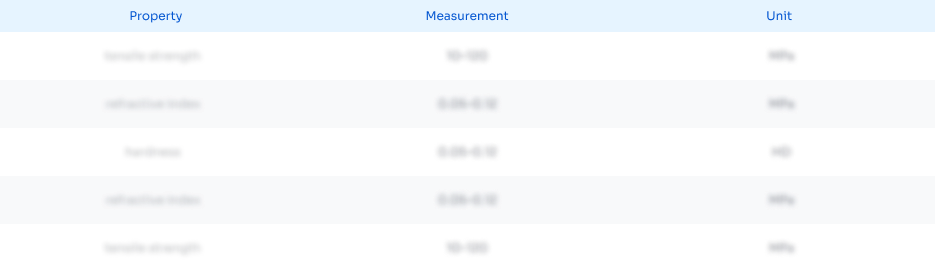
Abstract
Description
Claims
Application Information
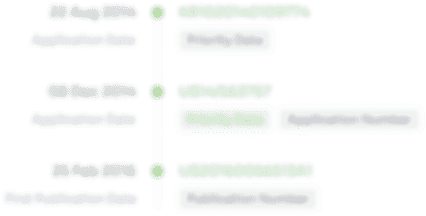
- R&D Engineer
- R&D Manager
- IP Professional
- Industry Leading Data Capabilities
- Powerful AI technology
- Patent DNA Extraction
Browse by: Latest US Patents, China's latest patents, Technical Efficacy Thesaurus, Application Domain, Technology Topic, Popular Technical Reports.
© 2024 PatSnap. All rights reserved.Legal|Privacy policy|Modern Slavery Act Transparency Statement|Sitemap|About US| Contact US: help@patsnap.com