A low-latency and high-reliability v2v resource allocation method based on deep reinforcement learning
A technology of reinforcement learning and resource allocation, applied in the field of Internet of Vehicles, it can solve the problems of not considering the energy consumption of V2V communication, unable to expand large-scale networks, and high transmission overhead, so as to maximize system energy efficiency, ensure reliability and delay requirements. , the effect of maximizing energy efficiency
- Summary
- Abstract
- Description
- Claims
- Application Information
AI Technical Summary
Problems solved by technology
Method used
Image
Examples
Embodiment Construction
[0036] The core idea of the present invention is: in order to make the communication between vehicles outside the coverage of the base station to meet the delay requirement while maximizing the energy efficiency, a low-latency and high-reliability V2V resource allocation method based on deep reinforcement learning is proposed .
[0037] The present invention will be described in further detail below.
[0038] Step (1), considering the area not covered by the base station, between vehicles (V2V) in order to transmit data related to driving safety, use URLLC slice resource blocks to communicate;
[0039]Step (2), the training phase, at each step, the V2V agent informs the computing unit of the current local observation information. The real environment state includes the global channel state and the behavior of all agents, which is agnostic to a single agent. Each V2V agent can only obtain part of the information that it can obtain, that is, observation information. The obse...
PUM
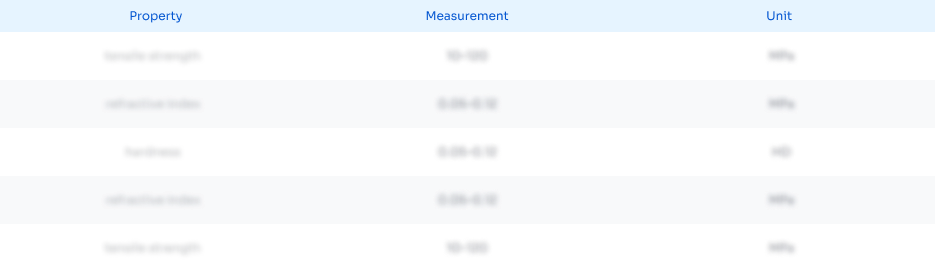
Abstract
Description
Claims
Application Information
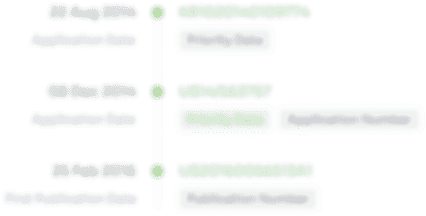
- R&D Engineer
- R&D Manager
- IP Professional
- Industry Leading Data Capabilities
- Powerful AI technology
- Patent DNA Extraction
Browse by: Latest US Patents, China's latest patents, Technical Efficacy Thesaurus, Application Domain, Technology Topic, Popular Technical Reports.
© 2024 PatSnap. All rights reserved.Legal|Privacy policy|Modern Slavery Act Transparency Statement|Sitemap|About US| Contact US: help@patsnap.com