Graph learning-based graph convolutional neural network traffic prediction method and system
A convolutional neural network and traffic forecasting technology, applied in the field of traffic forecasting, can solve problems such as the inability to capture multiple spatial relationships of the traffic network, insufficient capture of long-term time dependence, and single spatial relationship, so as to improve the accuracy of traffic forecasting and save training Effects of Time and Space Resources
- Summary
- Abstract
- Description
- Claims
- Application Information
AI Technical Summary
Problems solved by technology
Method used
Image
Examples
Embodiment Construction
[0080] The technical solutions in the present invention are clearly and completely described below in combination with the accompanying drawings in the embodiments of the present invention. Obviously, the described embodiments are only some of the embodiments of the present invention, not all of them. Based on the embodiments of the present invention, all other embodiments obtained by persons of ordinary skill in the art without creative efforts fall within the protection scope of the present invention.
[0081] A graph convolutional neural network traffic prediction method based on graph learning provided in this embodiment includes the following steps:
[0082] Step 1: Obtain the traffic speed data X of n historical moments of the predicted road section and its adjacent road sections n , where X n =[x 1 ,x 2 ,...,x k ,...,x n ],x k Indicates the traffic speed data at the kth historical moment.
[0083] Traffic forecasting is a time series forecasting problem, using hi...
PUM
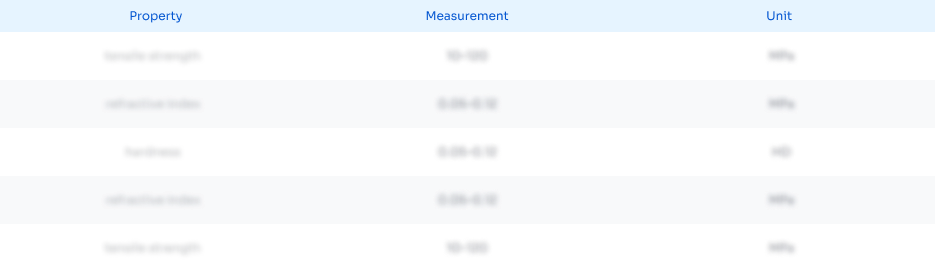
Abstract
Description
Claims
Application Information
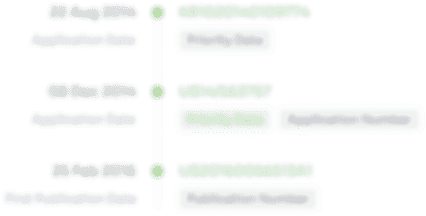
- R&D Engineer
- R&D Manager
- IP Professional
- Industry Leading Data Capabilities
- Powerful AI technology
- Patent DNA Extraction
Browse by: Latest US Patents, China's latest patents, Technical Efficacy Thesaurus, Application Domain, Technology Topic, Popular Technical Reports.
© 2024 PatSnap. All rights reserved.Legal|Privacy policy|Modern Slavery Act Transparency Statement|Sitemap|About US| Contact US: help@patsnap.com