Motor imagery electroencephalogram recognition method based on relative wavelet packet entropy brain network and improved lasso
A technology of motor imagery and recognition methods, applied in the field of EEG signals, can solve the problem of low accuracy of feature classification, and achieve the effect of reducing low accuracy and excellent features
- Summary
- Abstract
- Description
- Claims
- Application Information
AI Technical Summary
Problems solved by technology
Method used
Image
Examples
Embodiment 1
[0072] Such as figure 1 , this embodiment provides a motor imagery EEG recognition method based on relative wavelet packet entropy brain network and improved version of lasso, including steps S1-S3, specifically including:
[0073] S1. Data preprocessing, calculate R according to the power spectral density 2 Figure, obtain the frequency band with the largest amount of information in each data set and perform band-pass filtering, and perform channel screening through the SCSP algorithm, and filter the channels of each data set to obtain the optimal screening channel;
[0074] S2. Feature extraction, using the wavelet packet method to extract the detailed coefficients and approximate system of the EEG signal and calculate the wavelet packet energy entropy value to obtain the wavelet packet energy entropy feature, and construct the brain function network through the wavelet packet energy entropy value to extract brain function. The topological features of the network; and accord...
Embodiment 3
[0154] Such as Figure 8 , on the basis of Embodiment 1, this embodiment proposes a terminal device based on a relative wavelet packet entropy brain network and an improved version of lasso's motor imagery EEG recognition method. The terminal device 200 includes at least one memory 210 and at least one processor 220 and a bus 230 connecting different platform systems.
[0155] Memory 210 may include readable media in the form of volatile memory, such as random access memory (RAM) 211 and / or cache memory 212 , and may further include read only memory (ROM) 213 .
[0156] Wherein, the memory 210 also stores a computer program, and the computer program can be executed by the processor 220, so that the processor 220 executes any one of the above-mentioned motor imagery brain networks based on the relative wavelet packet entropy brain network and the improved lasso in the embodiment of the present application. The specific implementation of the electrical identification method is ...
Embodiment 4
[0161] On the basis of Embodiment 1, this embodiment proposes a computer-readable storage medium based on a relative wavelet packet entropy brain network and an improved version of lasso's motor imagery EEG recognition, and instructions are stored on the computer-readable storage medium, When the instruction is executed by the processor, any one of the above-mentioned motor imagery EEG recognition methods based on the relative wavelet packet entropy brain network and the improved version of lasso is realized. Its specific implementation mode is consistent with the implementation mode and achieved technical effect recorded in the above-mentioned method embodiments, and part of the content will not be repeated.
[0162] Figure 9 Shown is a program product 300 provided by this embodiment for realizing the above method, which may adopt a portable compact disk read-only memory (CD-ROM) and include program codes, and may run on a terminal device such as a personal computer. Howeve...
PUM
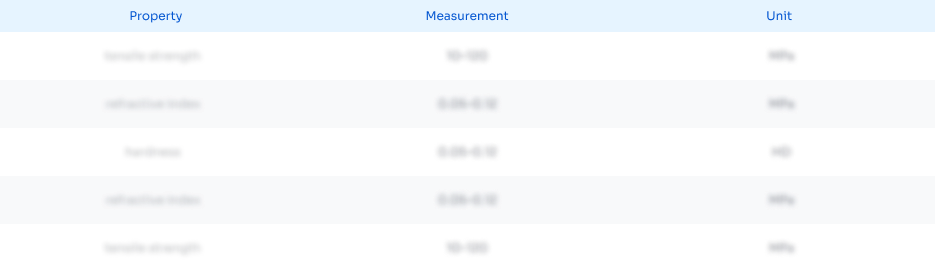
Abstract
Description
Claims
Application Information
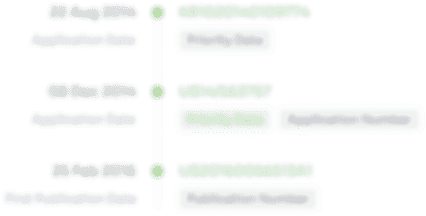
- Generate Ideas
- Intellectual Property
- Life Sciences
- Materials
- Tech Scout
- Unparalleled Data Quality
- Higher Quality Content
- 60% Fewer Hallucinations
Browse by: Latest US Patents, China's latest patents, Technical Efficacy Thesaurus, Application Domain, Technology Topic, Popular Technical Reports.
© 2025 PatSnap. All rights reserved.Legal|Privacy policy|Modern Slavery Act Transparency Statement|Sitemap|About US| Contact US: help@patsnap.com