Unsupervised pedestrian re-identification method based on hierarchical clustering and difficult sample triple
A pedestrian re-identification and hierarchical clustering technology, applied in character and pattern recognition, instruments, biological neural network models, etc., can solve the problems of low recognition accuracy and extraction of discriminative features, so as to reduce iteration cycle and improve accuracy , the effect of shortening the training time
- Summary
- Abstract
- Description
- Claims
- Application Information
AI Technical Summary
Problems solved by technology
Method used
Image
Examples
Embodiment 1
[0052] This embodiment provides an unsupervised person re-identification method based on hierarchical clustering and triple loss of difficult samples, such as figure 1 shown, including:
[0053] Step S1: Process the dataset and pre-allocate labels:
[0054] The image to be processed is divided into two parts, one part is the training set and the other part is the test set. Among them, the training set is the input for learning the CNN network, and the test set is the performance test and evaluation of the CNN network. When initializing the label of the training set, the image sequence number is used as the initial label, and the training set is set as X={x 1 ,x 2 ,...,x N},x i Represents the i-th image, where i=1,2,...,N, the initial label set is represents the image x i initial label for .
[0055] Step S2: Feature extraction:
[0056] Input all images of the training set assigned initial labels to the selected CNN model, for the i-th image x i Extract pedestrian...
PUM
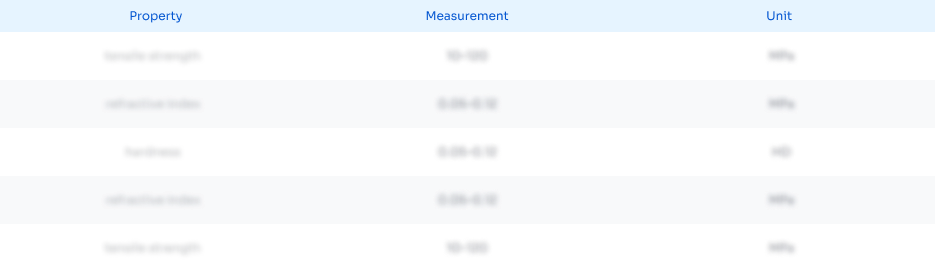
Abstract
Description
Claims
Application Information
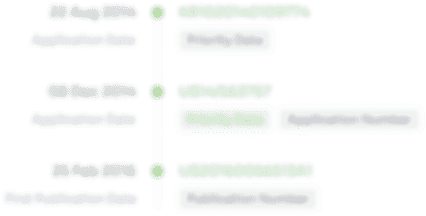
- R&D
- Intellectual Property
- Life Sciences
- Materials
- Tech Scout
- Unparalleled Data Quality
- Higher Quality Content
- 60% Fewer Hallucinations
Browse by: Latest US Patents, China's latest patents, Technical Efficacy Thesaurus, Application Domain, Technology Topic, Popular Technical Reports.
© 2025 PatSnap. All rights reserved.Legal|Privacy policy|Modern Slavery Act Transparency Statement|Sitemap|About US| Contact US: help@patsnap.com