A Click-through Rate Prediction Method Based on Multi-task Learning Mechanism
A multi-task learning and click-through rate technology, applied in the field of recommendation systems, can solve problems such as limited model combination capabilities, achieve good scalability and improve effectiveness
- Summary
- Abstract
- Description
- Claims
- Application Information
AI Technical Summary
Problems solved by technology
Method used
Image
Examples
Embodiment
[0051] When predicting the degree of interest of user U in question Q, the processing flow of the system is described as follows:
[0052] 1) Use Scikit-learn and Numpy tools to perform feature representation on user U and question Q, expressed as one-hot encoded features in the following form:
[0053]
[0054] 2) All features are densely represented by embedding pooling. Meta-Expert performs knowledge extraction on question Q, and MoE performs multi-angle portraits on user I and I's browsing question set.
[0055] 3) The output of each Expert module and Meta-Expert module in the MoE calculates the gating signal, through the weighted pooling of the gating signal, and finally obtains the user interest representation of the corresponding task.
[0056] 4) The CTR and CVR tasks target user interest representation vectors, and use logistic regression to output corresponding prediction results. The value range is [0,1]. A larger value indicates a greater degree of interest. ...
PUM
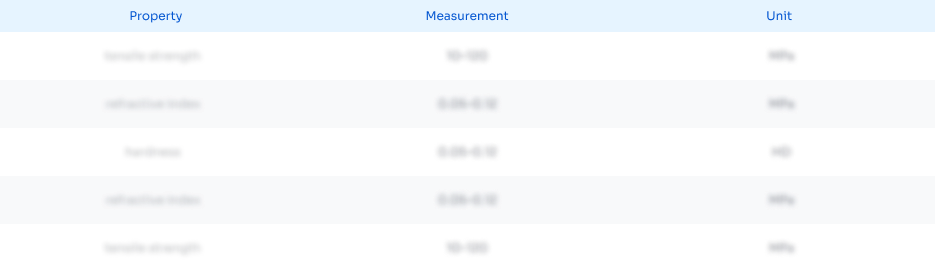
Abstract
Description
Claims
Application Information
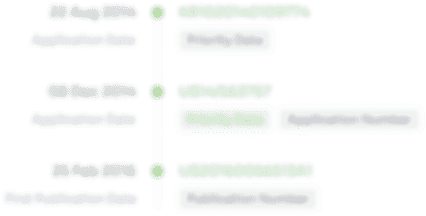
- R&D Engineer
- R&D Manager
- IP Professional
- Industry Leading Data Capabilities
- Powerful AI technology
- Patent DNA Extraction
Browse by: Latest US Patents, China's latest patents, Technical Efficacy Thesaurus, Application Domain, Technology Topic, Popular Technical Reports.
© 2024 PatSnap. All rights reserved.Legal|Privacy policy|Modern Slavery Act Transparency Statement|Sitemap|About US| Contact US: help@patsnap.com