Low-resolution image recognition algorithm for compensating edge information
A low-resolution image and high-resolution image technology, applied in the field of low-resolution image recognition algorithms, can solve the problems of large convolution kernel size, unsatisfactory recognition effect, and inability to effectively extract various features, so as to improve the recognition rate , Recognition algorithm robust effect
- Summary
- Abstract
- Description
- Claims
- Application Information
AI Technical Summary
Problems solved by technology
Method used
Image
Examples
Embodiment 1
[0040] The image recognition module of the present invention is mainly used for recognizing low-resolution images. This module uses the classic LeNet-5 model, which is an end-to-end network with a total of 7 layers, including 2 convolutional layers, 2 pooling layers and 3 fully connected layers. The parameters of each layer are shown in Table 1. . Traditional recognition networks directly convert low-resolution images to sent to the network for training, and the present invention is different from the traditional strategy, which uses low-resolution images and its estimated edge information image C pred After fusion, the input image is sent to the network for training, and the edge information image enhances the high-frequency detail information in the low-resolution image, so the recognition network can capture richer image features, thereby improving the recognition rate of low-resolution images .
[0041] Table 1 The parameters of each layer of LeNet-5 recognition netw...
Embodiment 2
[0044] The edge information generation module of the present invention is mainly used for estimating the edge information of the high-resolution image based on the low-resolution image. This module adopts the structure of generative confrontation network, which is divided into two parts: generation network and discriminant network. The specific network structure parameters are shown in Table 2.
[0045] Table 2 Network parameters of each layer of edge information generation module
[0046]
[0047]
[0048] The specific generation steps are as follows:
[0049] step1: input image preparation. The original high-resolution images in the training dataset I gt N times downsampling, and then N times upsampling to generate a low resolution image with the same size as the high resolution image Then use the Canny operator to extract the edge information of the high-resolution image and the low-resolution image respectively, and obtain the edge C of the high-resolution image...
Embodiment 3
[0057] The residual module containing the attention mechanism of the present invention is mainly used to select the features that are more critical to the prediction edge task from many features, and at the same time strengthen the anti-noise performance of the generation network. The specific network structure and its parameters are shown in Table 2.
[0058] Step1: Generate channel attention feature F1. The output features of the down-sampling module are sent to the feature extraction module to obtain the feature F1, and then the features are sent to the multi-layer perceptron with a hidden layer after passing through the maximum pooling layer and the average pooling layer, and then the The results are summed to obtain the channel attention feature F′.
[0059] step2: Generate the first correction feature F2. The channel attention feature F' is multiplied with the output feature of the feature extraction module to obtain the first modified feature F2.
[0060] step3: Gene...
PUM
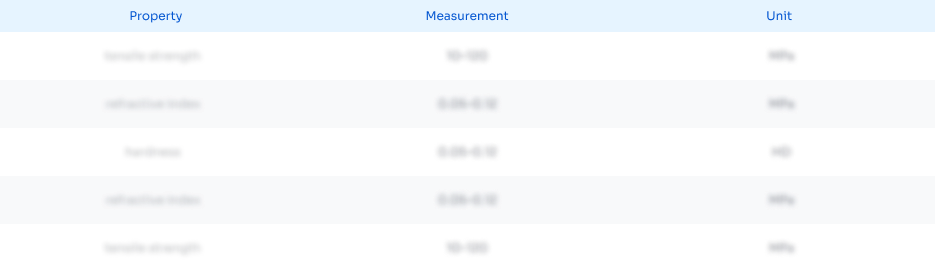
Abstract
Description
Claims
Application Information
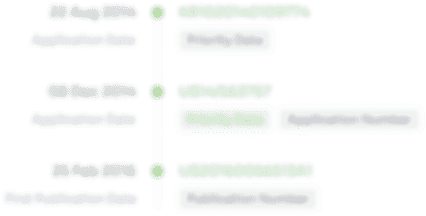
- R&D Engineer
- R&D Manager
- IP Professional
- Industry Leading Data Capabilities
- Powerful AI technology
- Patent DNA Extraction
Browse by: Latest US Patents, China's latest patents, Technical Efficacy Thesaurus, Application Domain, Technology Topic.
© 2024 PatSnap. All rights reserved.Legal|Privacy policy|Modern Slavery Act Transparency Statement|Sitemap