Medical image segmentation method and system based on distributed generative adversarial network
A medical image, distributed technology, applied in the field of medical image processing, can solve the problem of less training samples, and achieve the effect of reducing workload, reducing tedious operations, and saving use
- Summary
- Abstract
- Description
- Claims
- Application Information
AI Technical Summary
Problems solved by technology
Method used
Image
Examples
Embodiment 1
[0034] In this embodiment, a medical image segmentation method based on a distributed generation confrontation network is disclosed, including:
[0035] Set up a discriminator at each hospital, set up a generator on the central server, and build a generative confrontation network between each discriminator and generator;
[0036] Obtain medical images of various hospitals;
[0037] Generative adversarial networks are trained on medical images from various hospitals;
[0038] Segment the medical image to be segmented through the trained generative confrontation network.
[0039] Further, the medical images of each hospital include unlabeled medical images and manually labeled medical images.
[0040] Further, when training the generative confrontation network, random noise is input into the generator, and the generator generates a medical image fake image, and the medical image fake image and each medical image are input into each discriminator, and the unlabeled medical imag...
Embodiment 2
[0070] In this embodiment, a medical image segmentation system based on a distributed generation confrontation network is disclosed, including:
[0071] Generative confrontation network building blocks are used to set discriminators at each hospital, set generators on the central server, and build generative confrontation networks between discriminators and generators;
[0072] The medical image acquisition module is used to acquire the medical images of each hospital;
[0073] Generate a confrontation network training module, which is used to train the generation confrontation network through the medical images of each hospital;
[0074] The medical image segmentation module is used to segment the medical image to be segmented through the trained generation confrontation network.
Embodiment 3
[0076] In this embodiment, an electronic device is disclosed, including a memory, a processor, and computer instructions stored in the memory and executed on the processor. When the computer instructions are executed by the processor, the method disclosed in Embodiment 1 based on Steps described in Distributed Generative Adversarial Networks Method for Medical Image Segmentation.
PUM
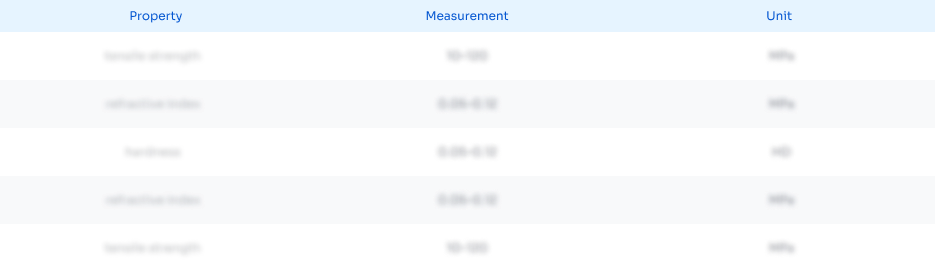
Abstract
Description
Claims
Application Information
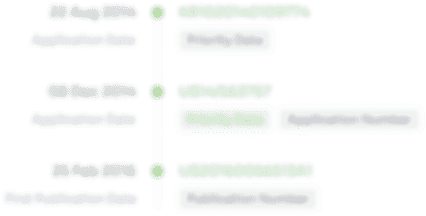
- R&D Engineer
- R&D Manager
- IP Professional
- Industry Leading Data Capabilities
- Powerful AI technology
- Patent DNA Extraction
Browse by: Latest US Patents, China's latest patents, Technical Efficacy Thesaurus, Application Domain, Technology Topic, Popular Technical Reports.
© 2024 PatSnap. All rights reserved.Legal|Privacy policy|Modern Slavery Act Transparency Statement|Sitemap|About US| Contact US: help@patsnap.com