WRSN multi-mobile charger optimal scheduling method based on reinforcement learning
A mobile charger, reinforcement learning technology, applied in specific environment-based services, current collectors, electric vehicles, etc., can solve problems such as lack of global optimality, node failure, modeling, solving and implementation difficulties, and achieve solutions. Local optimal problem, minimizing the number of dead nodes, maximizing the effect of charging utility
- Summary
- Abstract
- Description
- Claims
- Application Information
AI Technical Summary
Problems solved by technology
Method used
Image
Examples
Embodiment 1
[0057] A method for optimal dispatching of WRSN multi-mobile chargers based on reinforcement learning, comprising the following steps:
[0058] Step1: Build a wireless sensor network model, such as figure 1 Shown: N sensor nodes are randomly deployed in a certain area Ω, and the positions of the sensor nodes are all determined and known; these N sensor nodes are marked as O={o 1 , o 2 ,...,o n}, the battery power of the sensor node is b, and the energy consumption rate is P w J / s; the energy of the sensor node is mainly used to transmit data, when the node sends or receives a message of k bits, the energy consumption of the sensor node is as follows:
[0059]
[0060] where P elec Indicates the energy consumption per bit sent or received; d ij Indicates the distance between the sending node and the receiving node; μ indicates the energy consumption of the signal amplifier.
[0061] The M mobile chargers waiting to be dispatched are marked as C={c 1 , c 2 ,...,c m}...
PUM
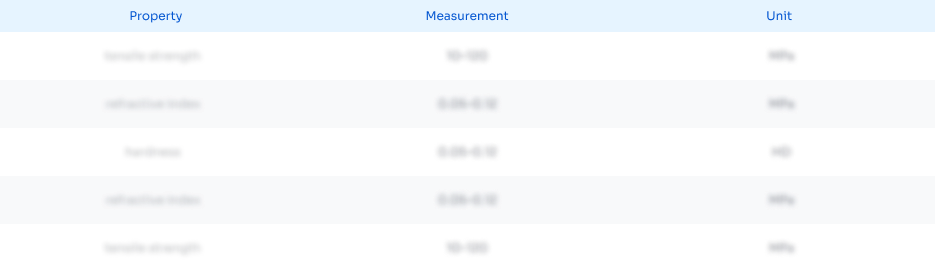
Abstract
Description
Claims
Application Information
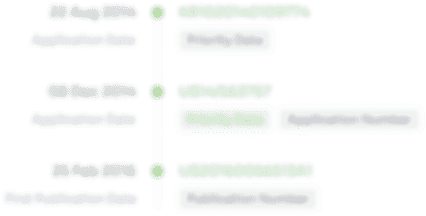
- R&D Engineer
- R&D Manager
- IP Professional
- Industry Leading Data Capabilities
- Powerful AI technology
- Patent DNA Extraction
Browse by: Latest US Patents, China's latest patents, Technical Efficacy Thesaurus, Application Domain, Technology Topic, Popular Technical Reports.
© 2024 PatSnap. All rights reserved.Legal|Privacy policy|Modern Slavery Act Transparency Statement|Sitemap|About US| Contact US: help@patsnap.com