A method and device for generating fluid density field based on deep learning
A technology of fluid density and deep learning, applied in neural learning methods, biological neural network models, instruments, etc., can solve problems such as low calculation accuracy, low resolution, and noise sensitivity
- Summary
- Abstract
- Description
- Claims
- Application Information
AI Technical Summary
Problems solved by technology
Method used
Image
Examples
Embodiment 1
[0104] 1. Generate a data set
[0105] The principle of data generation is as follows Figure 4 shown
[0106] (1) Construct a uniform density field 302 in advance, and perform different forms of heat source heating to change the density of the flow field under certain working conditions, and then use CFD simulation calculations to calculate a series of non-uniform density fields 306;
[0107] (2) Use zemax and other optical software to simulate the light paths of the light rays of the background pattern 301 passing through the uniform density field 302 and the above-mentioned non-uniform density field 306 with density gradients, as shown in figure 2 As shown, image sets are composed of background texture images at different camera positions at a preset camera position (the number of acquisition positions N≥2), and a uniform density background texture image set 303 and a non-uniform density background texture image set 305 are respectively obtained;
[0108] (3) Using the a...
Embodiment 2
[0116] The difference between the second embodiment and the first embodiment is that the input of the neural network and the generation of the data set are different.
[0117] The data set adopts the method of generating the second data set, and in step (3), an additional background pattern 301 is added as input to form the second data set.
[0118] The network structure of the second embodiment is as Figure 6 As shown, there is an input layer and an output layer. The input layer input image sets 303 and 305 and the image after the background pattern 301 are concatenated, its dimension is (256, 256, 7, 1), and then a set of 3D convolution is set The first layer extracts the features of the spliced images; after that, a set of deconvolution layers is set up to amplify the features, and finally the high-resolution and high-precision density field is restored through the output layer.
[0119] In practical applications, the two background texture image sets 303 and 305 and th...
Embodiment 3
[0122] 1. Generate a data set
[0123] The principle of data generation is as follows Figure 4 shown.
[0124] (1) Construct a uniform density field 302 in advance, and perform different forms of heat source heating to change the density of the flow field under certain working conditions, and then use CFD simulation calculations to calculate a series of non-uniform density fields 306;
[0125] (2) Use zemax and other optical software to simulate the light paths of the light rays of the background pattern 301 passing through the uniform density field 302 and the above-mentioned non-uniform density field 306 with density gradients, as shown in figure 2 As shown, image sets are composed of background texture images at different camera positions at a preset camera position (the number of acquisition positions N≥2), and a uniform density background texture image set 303 and a non-uniform density background texture image set 305 are respectively obtained;
[0126] (3) For the un...
PUM
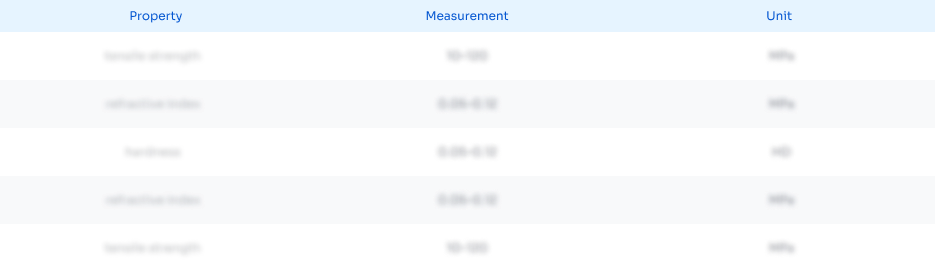
Abstract
Description
Claims
Application Information
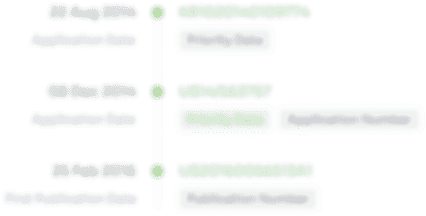
- R&D Engineer
- R&D Manager
- IP Professional
- Industry Leading Data Capabilities
- Powerful AI technology
- Patent DNA Extraction
Browse by: Latest US Patents, China's latest patents, Technical Efficacy Thesaurus, Application Domain, Technology Topic, Popular Technical Reports.
© 2024 PatSnap. All rights reserved.Legal|Privacy policy|Modern Slavery Act Transparency Statement|Sitemap|About US| Contact US: help@patsnap.com