Reinforcement learning method and related device
A technology of reinforcement learning and mutual influence, applied in the field of machine learning, it can solve the problems of low learning efficiency of complex strategies and achieve the effect of improving efficiency
- Summary
- Abstract
- Description
- Claims
- Application Information
AI Technical Summary
Problems solved by technology
Method used
Image
Examples
Embodiment Construction
[0037] As mentioned in the background technology, the methods for learning random strategies in the prior art mainly include methods such as soft actuator-evaluator (SoftActorCritic), soft Q-learning (SoftQ-learning) and path consistency learning (PathConsistency Learning). Both utilize a reinforcement learning framework based on entropy regularization. In this type of framework, the agent needs to maximize an additional entropy regularization term in addition to maximization. Commonly used entropy terms include Shannon entropy (Shannonentropy) and Tsallis entropy. The former can improve the sample efficiency of policy learning, that is, use fewer samples to learn better policies; while the solution using the latter is closer to the optimal solution of the original reinforcement learning problem.
[0038] However, entropy regularization often falls into a dilemma between simple policy representation and complex and inefficient training process. The general form of existing r...
PUM
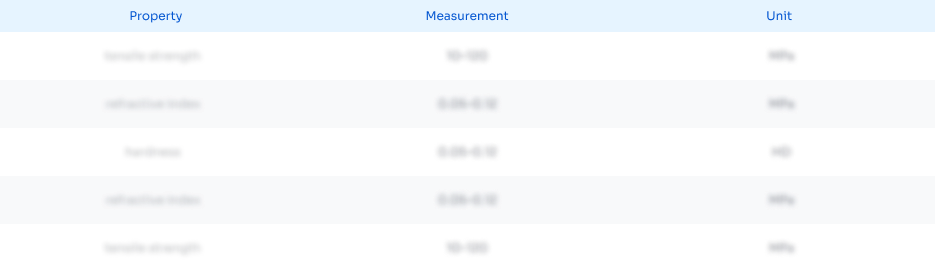
Abstract
Description
Claims
Application Information
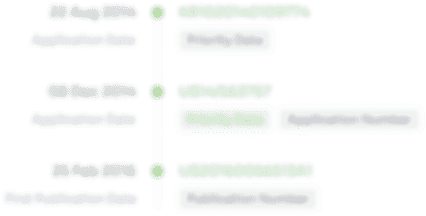
- Generate Ideas
- Intellectual Property
- Life Sciences
- Materials
- Tech Scout
- Unparalleled Data Quality
- Higher Quality Content
- 60% Fewer Hallucinations
Browse by: Latest US Patents, China's latest patents, Technical Efficacy Thesaurus, Application Domain, Technology Topic, Popular Technical Reports.
© 2025 PatSnap. All rights reserved.Legal|Privacy policy|Modern Slavery Act Transparency Statement|Sitemap|About US| Contact US: help@patsnap.com