Personalized recommendation method and system based on collaborative filtering and deep learning
A technology of deep learning and collaborative filtering, applied in neural learning methods, instruments, data processing applications, etc., can solve problems such as not fully utilizing information, ignoring collaborative signals, and not reflecting ideas, so as to improve accuracy and interpretability Effect
- Summary
- Abstract
- Description
- Claims
- Application Information
AI Technical Summary
Problems solved by technology
Method used
Image
Examples
Embodiment Construction
[0059] In order to make the purpose, technical solution and advantages of the present invention clearer, the technical solution of the present invention will now be described in detail in conjunction with the drawings and embodiments. It should be understood that the specific implementation cases described here are only used to explain the present invention, not to limit the present invention.
[0060] Such as figure 1 As shown, the personalized recommendation method based on collaborative filtering and deep learning provided by this embodiment is mainly divided into three stages: firstly, it is necessary to obtain the historical behavior data of the user's purchase of goods, perform preprocessing, and generate an implicit feedback interaction matrix. Then it is modeling a personalized recommendation system. This stage also includes obtaining the input vectors of users and products from the interaction matrix, and then generating hidden vectors of users and products respective...
PUM
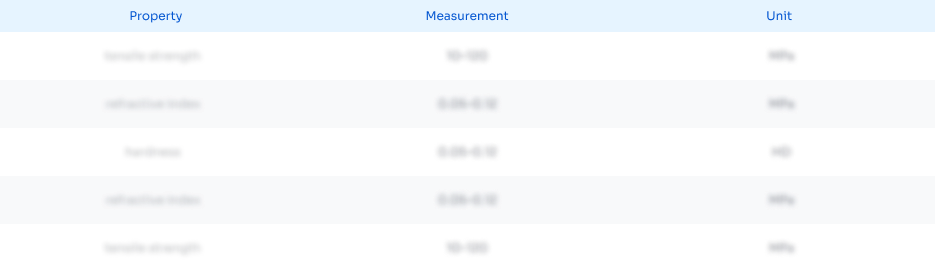
Abstract
Description
Claims
Application Information
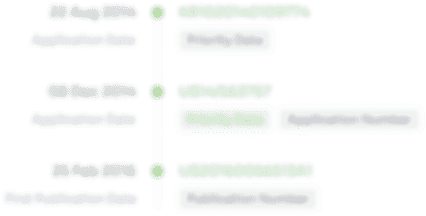
- R&D Engineer
- R&D Manager
- IP Professional
- Industry Leading Data Capabilities
- Powerful AI technology
- Patent DNA Extraction
Browse by: Latest US Patents, China's latest patents, Technical Efficacy Thesaurus, Application Domain, Technology Topic, Popular Technical Reports.
© 2024 PatSnap. All rights reserved.Legal|Privacy policy|Modern Slavery Act Transparency Statement|Sitemap|About US| Contact US: help@patsnap.com