Multi-modal emotion recognition method
An emotion recognition, multi-modal technology, applied in the field of data processing, can solve the problems of long sequence context modeling and other problems, achieve the effect of limited ability to solve and improve accuracy
- Summary
- Abstract
- Description
- Claims
- Application Information
AI Technical Summary
Problems solved by technology
Method used
Image
Examples
Embodiment 1
[0059] Such as figure 1 As shown, the multimodal emotion recognition method provided by the embodiment of the present application includes:
[0060] S1: Input the audio file, video file and corresponding text file of the sample to be tested, perform feature extraction on the audio file, video file and text file respectively, and obtain the audio feature at the frame level, the video feature at the frame level and the word level text features.
[0061] In some embodiments, the specific method for feature extraction of the audio file, video file and text file respectively includes:
[0062] Segmenting the audio file to obtain frame-level short-term audio clips; respectively inputting the short-time audio clips to a pre-trained audio feature extraction network to obtain the frame-level audio features;
[0063] Utilize face detection tool to extract the human face image of frame level from described video file; Input the human face image of described frame level into pre-trained...
Embodiment 2
[0110] The present application also discloses an electronic device, including a memory, a processor, and a computer program stored on the memory and operable on the processor. When the processor executes the computer program, the methods described in the above-mentioned embodiments are implemented. A step of.
Embodiment 3
[0112] The multimodal emotion recognition method includes the following steps:
[0113] S1-1: Input the audio to be tested, the video to be tested, and the text to be tested. The video and audio to be tested, the video to be tested, and the text to be tested are three different modalities.
[0114] In this embodiment, the audio to be tested and the video to be tested are video and audio in the same segment, the text to be tested corresponds to the audio to be tested and the video to be tested, and audio, video, and text are three types of video in this video modal.
[0115] In this embodiment, the data of these three modalities need to be analyzed in this embodiment to detect the emotional state of the character in the input segment.
[0116] According to the above scheme, further, a segment can be input, in which a character speaks, the continuous picture of this character speaking is the video to be tested, the audio that appears in the segment is the audio to be tested, th...
PUM
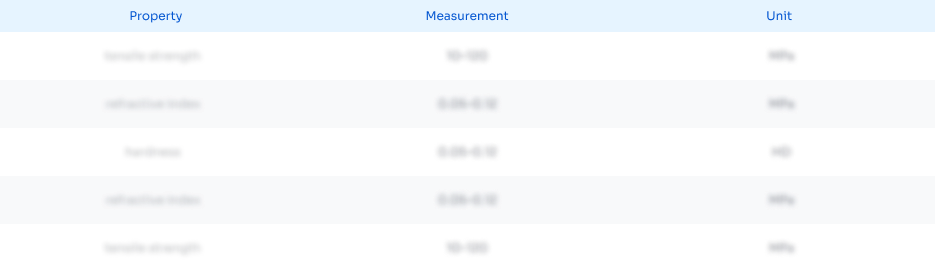
Abstract
Description
Claims
Application Information
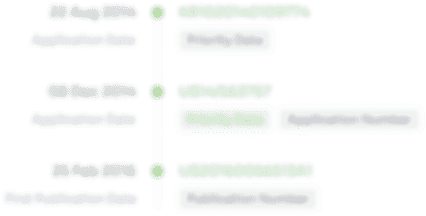
- R&D Engineer
- R&D Manager
- IP Professional
- Industry Leading Data Capabilities
- Powerful AI technology
- Patent DNA Extraction
Browse by: Latest US Patents, China's latest patents, Technical Efficacy Thesaurus, Application Domain, Technology Topic, Popular Technical Reports.
© 2024 PatSnap. All rights reserved.Legal|Privacy policy|Modern Slavery Act Transparency Statement|Sitemap|About US| Contact US: help@patsnap.com