Electromyographic signal noise reduction and classification method based on generative adversarial network
A technology of myoelectric signal and classification method, which is applied in the field of noise reduction and classification of myoelectric signal based on generative confrontation network, which can solve the problems of inaccurate response to muscle movement and inconvenience of human-computer interaction
- Summary
- Abstract
- Description
- Claims
- Application Information
AI Technical Summary
Problems solved by technology
Method used
Image
Examples
Embodiment Construction
[0033] The present invention will be described in detail below in conjunction with the accompanying drawings and specific embodiments.
[0034] Embodiments of the present invention provide a method for denoising and classifying EMG signals based on Generative Adversarial Networks, such as figure 1 As shown, the method is specifically implemented through the following steps:
[0035] Step 101: Build a WGAN-based generative adversarial network model for denoising EMG signals.
[0036] Specifically, the confrontation network model consists of a generation network and a discrimination network.
[0037] Input the noisy myoelectric signal to train the generation network, the noisy myoelectric signal is encoded and decoded by the generation network to generate the noise-reduced myoelectric signal, and then it is input to the discriminant network together with the noisy myoelectric signal, and compared with the noise-free myoelectric signal The electrical signals are compared, the l...
PUM
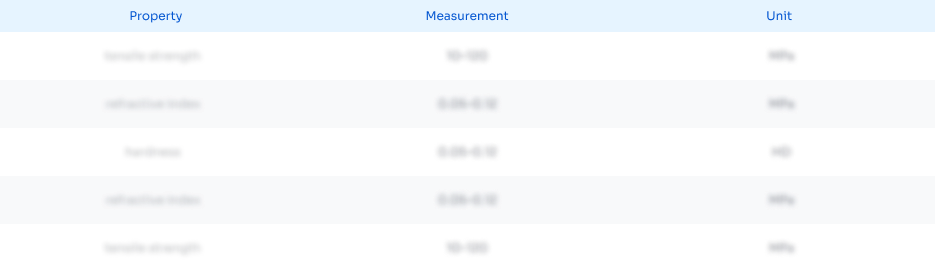
Abstract
Description
Claims
Application Information
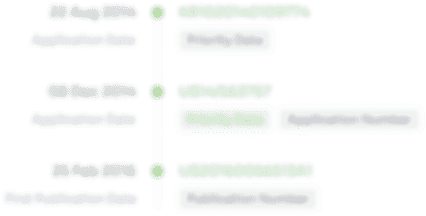
- R&D
- Intellectual Property
- Life Sciences
- Materials
- Tech Scout
- Unparalleled Data Quality
- Higher Quality Content
- 60% Fewer Hallucinations
Browse by: Latest US Patents, China's latest patents, Technical Efficacy Thesaurus, Application Domain, Technology Topic, Popular Technical Reports.
© 2025 PatSnap. All rights reserved.Legal|Privacy policy|Modern Slavery Act Transparency Statement|Sitemap|About US| Contact US: help@patsnap.com