Contour perception multi-organ segmentation network construction method based on class-by-class convolution operation
A multi-organ, convolution technology, applied in the fields of digital image processing, pattern recognition and medical imaging engineering, can solve problems such as higher effect requirements, 3D multi-organ segmentation optimization, and reduced complexity, so as to improve the convergence speed and enhance the capture. And the effect of restoring and reducing the corresponding loss
- Summary
- Abstract
- Description
- Claims
- Application Information
AI Technical Summary
Problems solved by technology
Method used
Image
Examples
Embodiment Construction
[0036] In order to better understand the technical solutions of the present invention, the implementation manners of the present invention will be further described below in conjunction with the accompanying drawings.
[0037] The present invention proposes a contour-aware multi-organ segmentation network construction method based on class-by-class convolution operations. Its network structure and algorithm framework are as follows: figure 1 As shown, the specific implementation details of each part are as follows:
[0038] Step 1: The multi-scale convolutional pyramid structure extracts three-dimensional features, outputs the rough segmentation results of multiple organs based on the upsampling region branch, and outputs the contour detection results of multiple organs based on the edge branch of the gated recurrent neural network. The specific method is as follows:
[0039] CT scan is composed of several continuous two-dimensional tomograms, and there is a close spatial rel...
PUM
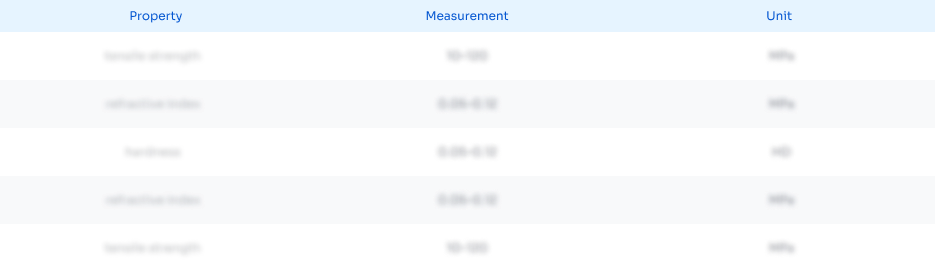
Abstract
Description
Claims
Application Information
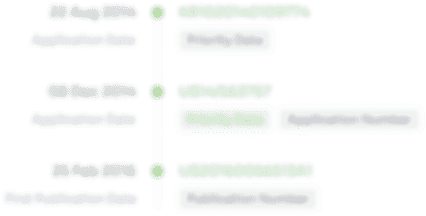
- R&D Engineer
- R&D Manager
- IP Professional
- Industry Leading Data Capabilities
- Powerful AI technology
- Patent DNA Extraction
Browse by: Latest US Patents, China's latest patents, Technical Efficacy Thesaurus, Application Domain, Technology Topic.
© 2024 PatSnap. All rights reserved.Legal|Privacy policy|Modern Slavery Act Transparency Statement|Sitemap