Ground penetrating radar roadbed disease target detection method based on convolutional neural network
A convolutional neural network and ground penetrating radar technology, applied in the field of ground penetrating radar subgrade disease target detection based on convolutional neural network, can solve problems such as spending a lot of energy and time, low detection efficiency, and lack of generalization ability.
- Summary
- Abstract
- Description
- Claims
- Application Information
AI Technical Summary
Problems solved by technology
Method used
Image
Examples
Embodiment Construction
[0093] The present invention will be described in further detail below in conjunction with the accompanying drawings.
[0094] see figure 1 As shown, the ground penetrating radar roadbed disease target detection method based on the convolutional neural network according to the present invention is operated and processed according to the following steps:
[0095] Step 1: Obtain the original image data of ground penetrating radar
[0096] Use the ground penetrating radar system to detect and collect the actual image data of the ground penetrating radar B-Scan, and use the FDTD-based gprMax software to perform forward modeling simulation on the three common types of diseases in the roadbed to generate the ground penetrating radar B-Scan simulation image ;
[0097] Step 2: GPR data preprocessing
[0098] The collected ground penetrating radar image data is normalized, zero bias removed, and the mean filter method is used to remove the direct wave and automatic gain processing, ...
PUM
Property | Measurement | Unit |
---|---|---|
Relative permittivity | aaaaa | aaaaa |
Abstract
Description
Claims
Application Information
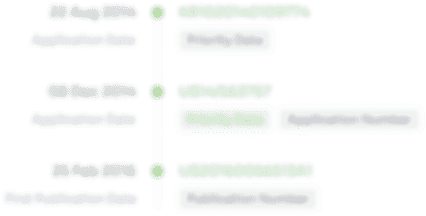
- Generate Ideas
- Intellectual Property
- Life Sciences
- Materials
- Tech Scout
- Unparalleled Data Quality
- Higher Quality Content
- 60% Fewer Hallucinations
Browse by: Latest US Patents, China's latest patents, Technical Efficacy Thesaurus, Application Domain, Technology Topic, Popular Technical Reports.
© 2025 PatSnap. All rights reserved.Legal|Privacy policy|Modern Slavery Act Transparency Statement|Sitemap|About US| Contact US: help@patsnap.com