Improved Bayesian adaptive resonance classification method
A classification method and Bayesian technology, applied in the field of neural networks, can solve problems such as difficult global convergence, classification performance degradation, and accuracy reduction, to achieve stable convergence, improve generalization ability, and prevent overfitting.
- Summary
- Abstract
- Description
- Claims
- Application Information
AI Technical Summary
Problems solved by technology
Method used
Image
Examples
Embodiment Construction
[0050]The following will clearly and completely describe the technical solutions in the embodiments of the present invention with reference to the accompanying drawings in the embodiments of the present invention. Obviously, the described embodiments are only some, not all, embodiments of the present invention. Based on the embodiments of the present invention, all other embodiments obtained by persons of ordinary skill in the art without making creative efforts belong to the protection scope of the present invention.
[0051] The embodiment of the present invention discloses an improved Bayesian adaptive resonance classification method, comprising the following steps:
[0052] S1. Construct the RQ-BR-BAM model, and introduce a rational quadratic kernel function, the overfitting suppression mechanism of RQ, and Bayesian regularization, the overfitting suppression mechanism of BR
[0053] S2, preprocessing the data, and setting test parameters and RQ-BR-BAM model parameters;
...
PUM
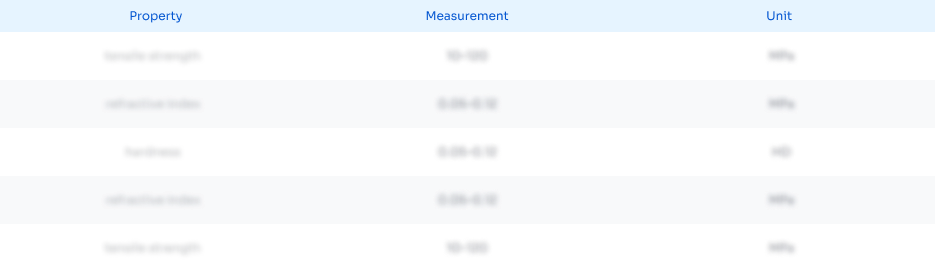
Abstract
Description
Claims
Application Information
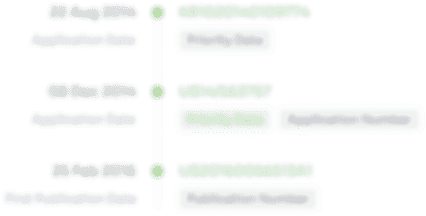
- R&D Engineer
- R&D Manager
- IP Professional
- Industry Leading Data Capabilities
- Powerful AI technology
- Patent DNA Extraction
Browse by: Latest US Patents, China's latest patents, Technical Efficacy Thesaurus, Application Domain, Technology Topic.
© 2024 PatSnap. All rights reserved.Legal|Privacy policy|Modern Slavery Act Transparency Statement|Sitemap