Intermittent process fault identification method and system based on TDKSFA nonlinear contribution graph
A fault identification, non-linear technology, applied in chemical process analysis/design, chemical statistics, character and pattern recognition, etc., can solve the problem of reducing fault diagnosis performance, unable to identify the type of fault data to be identified, unable to handle intermittent process two at the same time. dimensional dynamic characteristics and other issues to achieve the effect of improving accuracy and performance
- Summary
- Abstract
- Description
- Claims
- Application Information
AI Technical Summary
Problems solved by technology
Method used
Image
Examples
Embodiment 1
[0068] This embodiment takes the penicillin fermentation process as an example. The penicillin fermentation process is a well-known benchmark simulation process widely used to evaluate fault detection methods for batch processes.
[0069] The schematic diagram of the penicillin fermentation process is shown in image 3 , the fermentation process consists of two operational stages: a pre-cultivation stage and a fed-batch stage. During the initial pre-incubation phase, the nutrients necessary for a large number of cells begin to be produced and penicillin cells emerge during the exponential growth phase of the cells. In the fed-batch phase, in order to maintain a high penicillin production, it is necessary to continuously supply glucose to the fermentation process to keep the biomass growth rate constant. In order to provide the best conditions for the production of penicillin, closed-loop control of the temperature and pH of the fermenter is used.
[0070] In the simulation e...
Embodiment 2
[0176] This embodiment provides a batch process fault identification system based on the TDKSFA nonlinear contribution graph, which includes:
[0177] The non-linear contribution calculation module is used to perform mean centering operations on the pseudo-sample kernel matrices corresponding to the fault data set to be identified and the average training data set, and respectively project them to the load matrix of the TDKSFA model to obtain the corresponding score matrix, according to The difference between the two pseudo-sample projection trajectories, and then define the nonlinear contribution of any process variable relative to the fault, and calculate the nonlinear contribution of all process variables relative to the fault;
[0178] A fault type identification module, which is used to identify the fault variable according to the magnitude of the nonlinear contribution of each process variable, and determine the fault type of the fault data to be identified;
[0179] Amo...
Embodiment 3
[0184] This embodiment provides a computer-readable storage medium, on which a computer program is stored. When the program is executed by a processor, the steps in the intermittent process fault identification method based on the TDKSFA nonlinear contribution graph as described in the first embodiment above are realized. step.
PUM
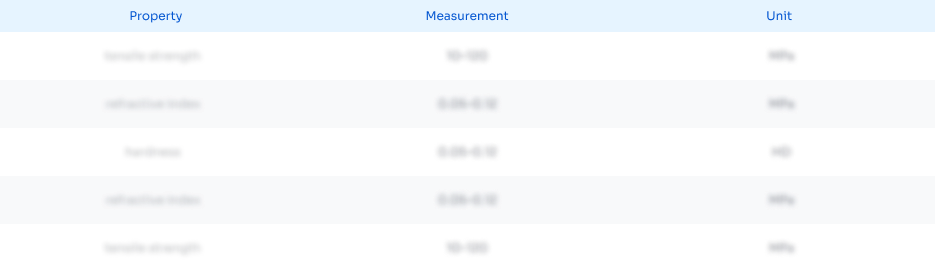
Abstract
Description
Claims
Application Information
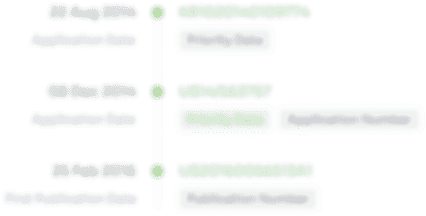
- R&D Engineer
- R&D Manager
- IP Professional
- Industry Leading Data Capabilities
- Powerful AI technology
- Patent DNA Extraction
Browse by: Latest US Patents, China's latest patents, Technical Efficacy Thesaurus, Application Domain, Technology Topic.
© 2024 PatSnap. All rights reserved.Legal|Privacy policy|Modern Slavery Act Transparency Statement|Sitemap