An adaptive traffic signal control method based on multi-agent reinforcement learning
A technology of reinforcement learning and traffic signals, applied in the traffic control system of road vehicles, traffic control systems, instruments, etc., can solve problems such as incoordination, instability, and difficulty in learning cooperative strategies, so as to reduce the difficulty of training , improve the probability, reduce the effect of time delay and overhead
- Summary
- Abstract
- Description
- Claims
- Application Information
AI Technical Summary
Problems solved by technology
Method used
Image
Examples
Embodiment Construction
[0034] The present invention establishes a weak collaborative traffic model that uses independent learning agent to control the phase selection of traffic lights of each intersection, which can observe the road conditions of the intersection. The traffic model uses a simple state definition and reduces the optimization target of the independent smart body to a local area including the neighbor intersection. In response to this model, the present invention proposes a separate collaborative enhancement learning algorithm-Cooperative IMDEPENT LENIENTDOUBLE DQN (CIL-DDQN), which borrows independent Q-Learning and a large degree of idea on the DDQN algorithm to improve independent intelligence The coordination ability is between. The specific innovation of the algorithm is mainly in the following two aspects: First, forgetful experience pool, the stored experience is composed of two parts: the importance of experience and experience; the second, the algorithm loss function is defined a...
PUM
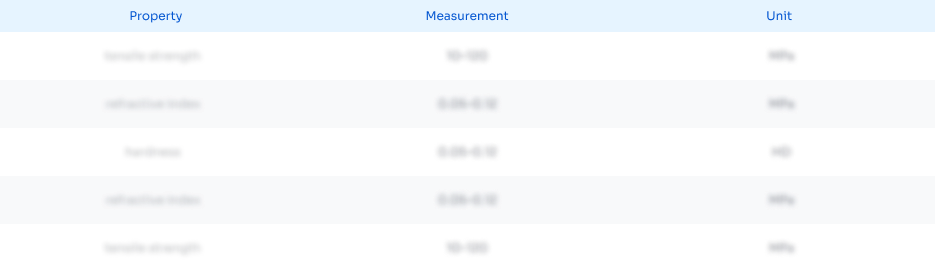
Abstract
Description
Claims
Application Information
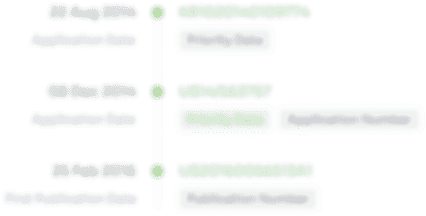
- R&D
- Intellectual Property
- Life Sciences
- Materials
- Tech Scout
- Unparalleled Data Quality
- Higher Quality Content
- 60% Fewer Hallucinations
Browse by: Latest US Patents, China's latest patents, Technical Efficacy Thesaurus, Application Domain, Technology Topic, Popular Technical Reports.
© 2025 PatSnap. All rights reserved.Legal|Privacy policy|Modern Slavery Act Transparency Statement|Sitemap|About US| Contact US: help@patsnap.com