Regression modeling method based on regression attention generative adversarial network data enhancement
A modeling method and network data technology, applied in the field of regression modeling, can solve problems such as restricting the effect of data enhancement, and achieve the effect of improving performance and prediction accuracy
- Summary
- Abstract
- Description
- Claims
- Application Information
AI Technical Summary
Problems solved by technology
Method used
Image
Examples
Embodiment Construction
[0031] The regression modeling method of the present invention based on regression attention generation and confrontation network data enhancement will be further described below in conjunction with specific embodiments.
[0032] The Regression Attention Generative Adversarial Network (RA-GAN) proposed by the present invention draws on the basic structure of the Wasserstein network with a gradient penalty term, and introduces two attention modules, namely the attention module 1 in the generator and the discriminator The attention module in 2. The independent variable in the present invention is the process variable in the industrial process, and the dependent variable is the corresponding quality variable.
[0033] Such as figure 1 As shown, the generator of Regression Attention Generative Adversarial Network (RA-GAN) is a multi-layer perceptron, the input layer input is random noise z, the setting of hidden layer is [32,32], and the output layer outputs generated data D'=[X...
PUM
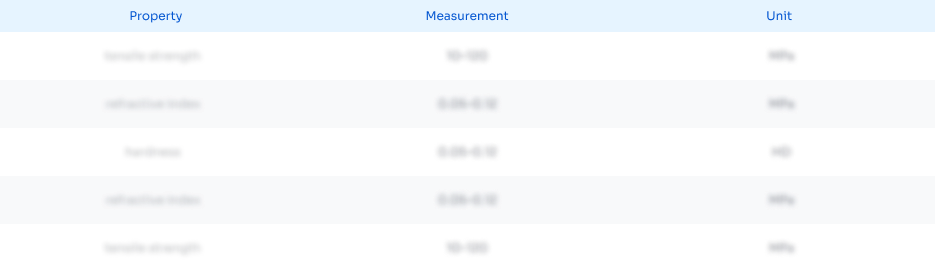
Abstract
Description
Claims
Application Information
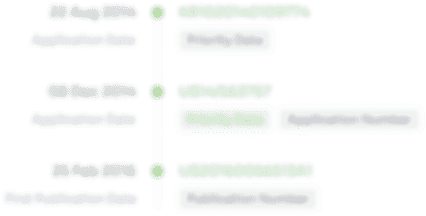
- Generate Ideas
- Intellectual Property
- Life Sciences
- Materials
- Tech Scout
- Unparalleled Data Quality
- Higher Quality Content
- 60% Fewer Hallucinations
Browse by: Latest US Patents, China's latest patents, Technical Efficacy Thesaurus, Application Domain, Technology Topic, Popular Technical Reports.
© 2025 PatSnap. All rights reserved.Legal|Privacy policy|Modern Slavery Act Transparency Statement|Sitemap|About US| Contact US: help@patsnap.com