Image three-dimensional tissue segmentation and determination method based on deep neural network
A technology of deep neural network and measurement method, applied in the field of image 3D tissue segmentation and measurement based on deep neural network, to achieve the effect of 3D tissue segmentation and measurement
- Summary
- Abstract
- Description
- Claims
- Application Information
AI Technical Summary
Problems solved by technology
Method used
Image
Examples
Embodiment 1
[0061] refer to figure 1 , which is the first embodiment of the present invention, this embodiment provides a method for image three-dimensional tissue segmentation and measurement based on a deep neural network, including:
[0062] S1: Collect CT images of live pigs, divide them into training set and test set, and mark them on the training set.
[0063] S2: Construct a CT bed segmentation network and a viscera segmentation network, and use the marked training set to train to obtain a CT bed segmentation model and a viscera segmentation model. It should be noted that,
[0064] The CT bed segmentation network is mainly composed of an encoder and a decoder. The encoder includes three sub-modules, and each sub-module includes two a×a convolution operations, a residual block, and a b×b maximum pooling; The encoder consists of three sub-modules, each of which consists of a c×c deconvolution, two d×d convolution operations and a residual block, where each convolution operation in ...
Embodiment 2
[0099] refer to Figure 2-9 , is the second embodiment of the present invention, after selecting 40 healthy breeding pigs to inject sedatives, the position of the breeding pigs is fixed in a way of separating the limbs and sent into the CT machine, and the CT images are taken, and the CT images of a breeding pig are The image is regarded as a three-dimensional array T(x, y, z), and finally 40 three-dimensional arrays are generated to obtain CT image slices, and the image data of 10 breeding pigs are selected as the training set, and the image sets of the remaining 30 pigs are used as Test set, and manually mark the CT bed and pig's viscera on the training set; train the viscera segmentation network with the marked viscera training set, obtain the viscera segmentation model, and use the CT bed segmentation model to predict the CT bed mask map on the test set , and remove the CT bed from the original image, and extract the fat, muscle, and bone parts of the pig body based on the...
PUM
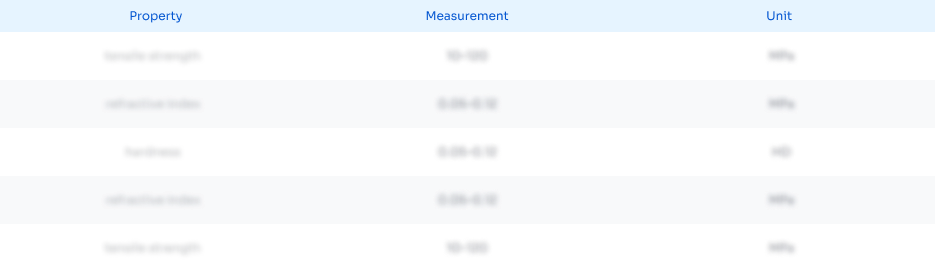
Abstract
Description
Claims
Application Information
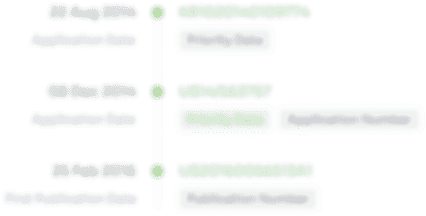
- Generate Ideas
- Intellectual Property
- Life Sciences
- Materials
- Tech Scout
- Unparalleled Data Quality
- Higher Quality Content
- 60% Fewer Hallucinations
Browse by: Latest US Patents, China's latest patents, Technical Efficacy Thesaurus, Application Domain, Technology Topic, Popular Technical Reports.
© 2025 PatSnap. All rights reserved.Legal|Privacy policy|Modern Slavery Act Transparency Statement|Sitemap|About US| Contact US: help@patsnap.com