Coating defect detection method based on deep learning
A technology of deep learning and defect detection, applied in neural learning methods, image data processing, image enhancement, etc., can solve problems such as missed detection and false detection, and inability to detect online in real time
- Summary
- Abstract
- Description
- Claims
- Application Information
AI Technical Summary
Problems solved by technology
Method used
Image
Examples
Embodiment Construction
[0021] The following will clearly and completely describe the technical solutions in the embodiments of the present invention with reference to the accompanying drawings in the embodiments of the present invention. Obviously, the described embodiments are only some, not all, embodiments of the present invention. Based on the embodiments of the present invention, all other embodiments obtained by persons of ordinary skill in the art without making creative efforts belong to the protection scope of the present invention.
[0022] see figure 1 , the present invention is a coating defect detection system based on deep learning, specifically comprising:
[0023] The image acquisition module 10 is used to obtain images of various types of melt-blown cloth coating surface of the coating production line; the image acquisition module 10 adopts two high-resolution industrial CMOS cameras, and the two cameras are vertically aligned with the film material Specifically, LEDs can also be u...
PUM
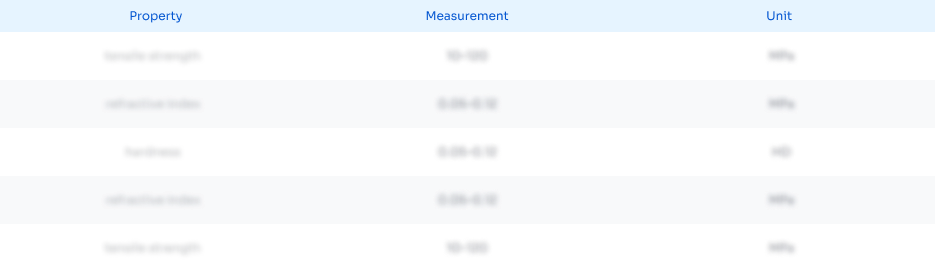
Abstract
Description
Claims
Application Information
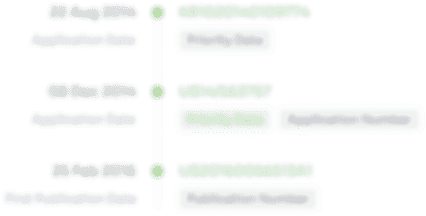
- R&D
- Intellectual Property
- Life Sciences
- Materials
- Tech Scout
- Unparalleled Data Quality
- Higher Quality Content
- 60% Fewer Hallucinations
Browse by: Latest US Patents, China's latest patents, Technical Efficacy Thesaurus, Application Domain, Technology Topic, Popular Technical Reports.
© 2025 PatSnap. All rights reserved.Legal|Privacy policy|Modern Slavery Act Transparency Statement|Sitemap|About US| Contact US: help@patsnap.com