Copula and stacked LSTM network coupled park multivariate load joint prediction method coupled with
A forecasting method and stacked technology, applied in forecasting, biological neural network models, data processing applications, etc.
- Summary
- Abstract
- Description
- Claims
- Application Information
AI Technical Summary
Problems solved by technology
Method used
Image
Examples
Embodiment 1
[0045] refer to figure 1 , figure 2 and image 3 , which is the first embodiment of the present invention, provides a multi-element load joint forecasting method for parks that couples Copula and stacked LSTM networks, including:
[0046] S1: Collect historical data of cooling loads, heating loads, and electrical loads in typical seasons in the park, as well as temperature data and holiday information at corresponding time nodes. What needs to be explained is:
[0047] Collect historical data such as typical seasonal (summer) cooling load and electrical load, typical seasonal (winter) heating load and electrical load;
[0048] Collect temperature data and holiday information on corresponding time nodes;
[0049] Among them, the time resolution of cold, heat, and electric loads is 15 minutes, and the temperature data is the highest temperature throughout the day.
[0050] S2: Perform noise detection and repair on historical data and normalize data in combination with temp...
Embodiment 2
[0092] In order to better verify and explain the technical effects adopted in the method of the present invention, this embodiment chooses to compare and test the traditional two-dimensional output method of the classic LSTM model with the method of the present invention, and compare the test results by means of scientific demonstration to verify the present invention. The real effect of the invented method.
[0093] refer to Figure 4 , which is the second embodiment of the present invention. This embodiment is different from the first embodiment in that it provides a prediction accuracy verification of a park multi-element load joint prediction method that couples Copula and stacked LSTM networks, including:
[0094] The stacked LSTM network is to pass the information flow solved by each LSTM layer to the next layer, and provide output at the last layer. The stacking mechanism deepens the extraction of sequence data information features. The stacked LSTM model framework is c...
PUM
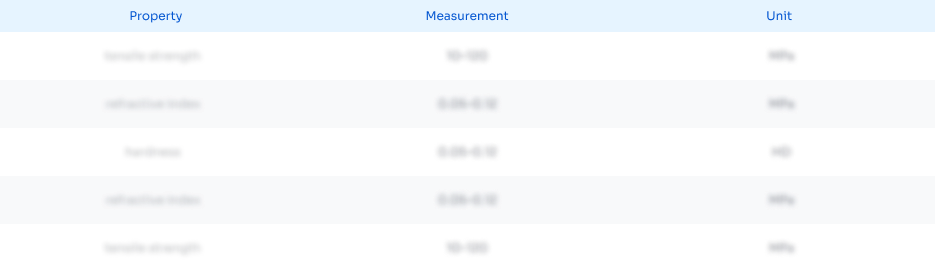
Abstract
Description
Claims
Application Information
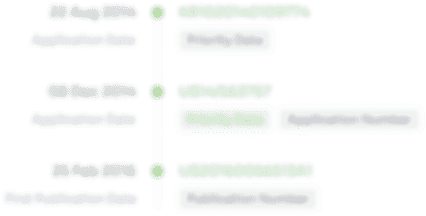
- R&D Engineer
- R&D Manager
- IP Professional
- Industry Leading Data Capabilities
- Powerful AI technology
- Patent DNA Extraction
Browse by: Latest US Patents, China's latest patents, Technical Efficacy Thesaurus, Application Domain, Technology Topic, Popular Technical Reports.
© 2024 PatSnap. All rights reserved.Legal|Privacy policy|Modern Slavery Act Transparency Statement|Sitemap|About US| Contact US: help@patsnap.com