Few-time text classification method under meta-learning framework based on measurement
A text classification and meta-learning technology, which is applied in text database clustering/classification, text database query, unstructured text data retrieval, etc., can solve the problems of indistinguishable and ignoring prototypes
- Summary
- Abstract
- Description
- Claims
- Application Information
AI Technical Summary
Problems solved by technology
Method used
Image
Examples
Embodiment Construction
[0066] The present invention will be further described below in conjunction with the accompanying drawings, but the present invention is not limited in any way. Any transformation or replacement based on the teaching of the present invention belongs to the protection scope of the present invention.
[0067] Such as figure 1 As shown, the few-shot text classification method based on the metric-based meta-learning framework includes the following steps:
[0068] Step 1, in the input layer, input support instance and query instance;
[0069] Step 2, in the word embedding layer, by looking up the pre-trained word embedding table, the discrete words of the support instance and the query instance are mapped into the vector space;
[0070] Step 3, at the contextual encoder layer, optimize the local representation of each word in the sentence of the supporting instance and the query instance by considering the context;
[0071] Step 4, in the bidirectional attention layer, the query...
PUM
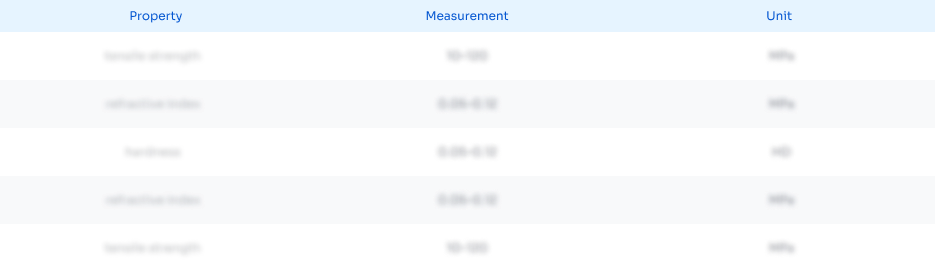
Abstract
Description
Claims
Application Information
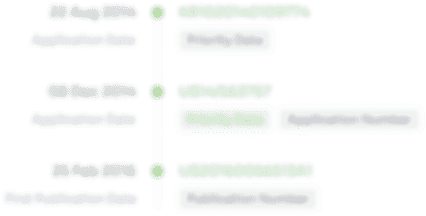
- Generate Ideas
- Intellectual Property
- Life Sciences
- Materials
- Tech Scout
- Unparalleled Data Quality
- Higher Quality Content
- 60% Fewer Hallucinations
Browse by: Latest US Patents, China's latest patents, Technical Efficacy Thesaurus, Application Domain, Technology Topic, Popular Technical Reports.
© 2025 PatSnap. All rights reserved.Legal|Privacy policy|Modern Slavery Act Transparency Statement|Sitemap|About US| Contact US: help@patsnap.com