Small sample picture classification model and method based on semantic auxiliary attention mechanism
A technology of image classification and sample images, applied in computer parts, character and pattern recognition, instruments, etc., can solve the problems of areas susceptible to the influence of the external environment, ambiguity, and classification tasks, and achieve good classification of small sample images. , the effect of improving performance
- Summary
- Abstract
- Description
- Claims
- Application Information
AI Technical Summary
Problems solved by technology
Method used
Image
Examples
Embodiment
[0076] In the embodiment of the present invention, a small-sample image classification model based on a semantic-assisted attention mechanism is taken as an example to describe in detail. The overall structure of the module is as follows figure 2 shown. The module consists of two branches: a spatial attention mechanism and a semantic alignment mechanism. Suppose our input feature map is The module aims to generate masks for it, that is, a semantically assisted attention map Therefore, suppose the output feature map is Calculate according to the following formula:
[0077]
[0078] in, is a bitwise multiplication operation that applies the attention value to the input feature map.
[0079] The spatial attention mechanism aims to mine the internal spatial associations of the features of the input feature map. In other words, it helps the model determine where to focus on the input feature map, highlights key regional features in the input feature map, suppresses u...
PUM
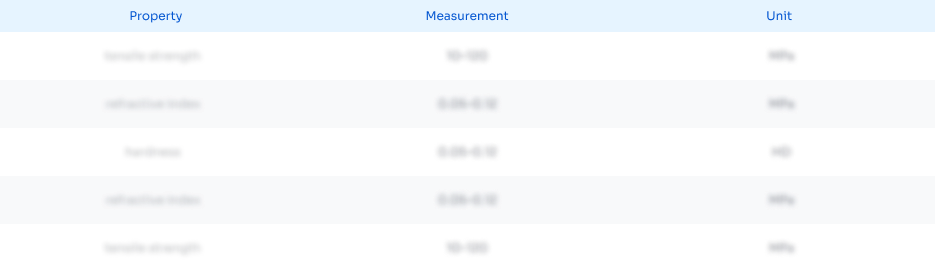
Abstract
Description
Claims
Application Information
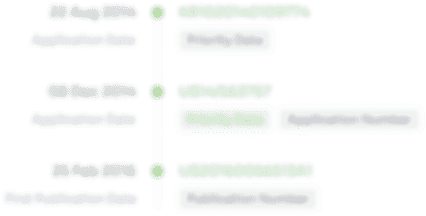
- R&D Engineer
- R&D Manager
- IP Professional
- Industry Leading Data Capabilities
- Powerful AI technology
- Patent DNA Extraction
Browse by: Latest US Patents, China's latest patents, Technical Efficacy Thesaurus, Application Domain, Technology Topic, Popular Technical Reports.
© 2024 PatSnap. All rights reserved.Legal|Privacy policy|Modern Slavery Act Transparency Statement|Sitemap|About US| Contact US: help@patsnap.com