Aircraft assembly production line productivity prediction method based on deep neural network
A deep neural network and production capacity forecasting technology, which is applied in the field of production capacity forecasting of aircraft assembly lines, can solve problems such as cumbersome operations, inability to analyze resource allocation schemes, and long time, and achieve the effects of low computing resource requirements, good adaptability, and promotional effects
- Summary
- Abstract
- Description
- Claims
- Application Information
AI Technical Summary
Problems solved by technology
Method used
Image
Examples
Embodiment Construction
[0035] The present invention will be described in detail below in conjunction with specific embodiments. The following examples will help those skilled in the art to further understand the present invention, but do not limit the present invention in any form. It should be noted that those skilled in the art can make several changes and improvements without departing from the concept of the present invention. These all belong to the protection scope of the present invention.
[0036] The invention proposes a method for predicting the production capacity of an aircraft assembly line based on a deep neural network. Firstly, different types of resources and production capacity are selected as variables, and the corresponding resource allocation plan and historical production capacity change value are obtained from the historical data of the production line. One part is used for DNN model training (training data set), and the other part is used to test the prediction accuracy of D...
PUM
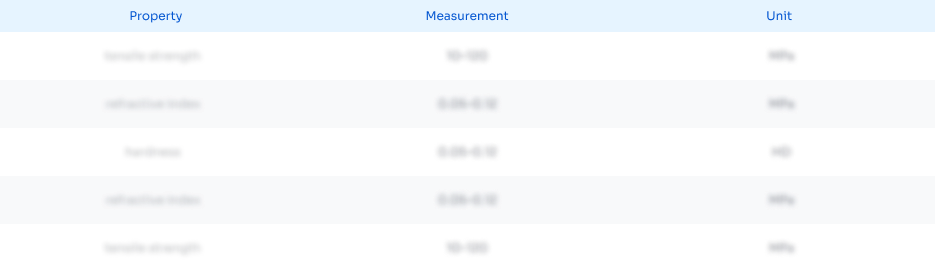
Abstract
Description
Claims
Application Information
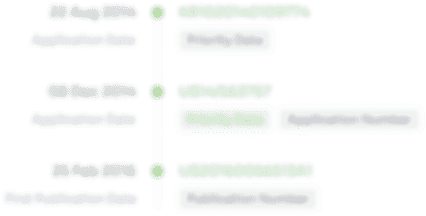
- R&D Engineer
- R&D Manager
- IP Professional
- Industry Leading Data Capabilities
- Powerful AI technology
- Patent DNA Extraction
Browse by: Latest US Patents, China's latest patents, Technical Efficacy Thesaurus, Application Domain, Technology Topic, Popular Technical Reports.
© 2024 PatSnap. All rights reserved.Legal|Privacy policy|Modern Slavery Act Transparency Statement|Sitemap|About US| Contact US: help@patsnap.com