Feature selection method based on self-adaptive LASSO
A feature selection method, an adaptive technique, used in mathematics and computer science to solve problems such as the inability to guarantee feature consistency
- Summary
- Abstract
- Description
- Claims
- Application Information
AI Technical Summary
Problems solved by technology
Method used
Image
Examples
Embodiment 1
[0079] The data in this example comes from The Cancer Genome Atlas (TCGA) database, using the methylation expression data of liver cancer cells, where the cancer samples are taken from the cells of cancer organs, and the normal samples are taken from organs at a certain distance from the cancer organs in the cell. The dimension of the data set is 485577, and the number of samples is 100, including 50 cancer samples and 50 normal samples. According to the proportion of 70% training set and 30% test set, the data set is divided into two parts, and the feature selection method is implemented on the training set. Firstly, the Student's t-test is performed on the training data, and 1000 features with the smallest p value are selected; then, this method is implemented on the 1000 feature data for feature selection, and 8 features are screened. These 8 features and 1000 features were used to train the linear SVM model to verify the test set, and finally the same classification accur...
Embodiment 2
[0081] The data in this example comes from the Uci Machine Learning Repository, using the Sentiment Labeled Sentences Data Set. The data is randomly sampled from Amazon's shopping reviews to determine whether the reviews are positive or not. The data set has 1000 samples, including 500 positive samples and 500 negative samples. The text data is vectorized through the bag of words model to obtain 1897-dimensional training data. According to the proportion of 70% training set and 30% test set, the data set is divided into two parts, and the feature selection method is implemented on the training set. Because this data set is discretized data, the Relief algorithm cannot be used to calculate the degree of difference between the same and different classes, so only the adaptive Lasso method with symmetric uncertainty is used for feature selection, and 224 features are screened. The 224 features and 1897 features were used to train the linear SVM model to verify the test set, and ...
PUM
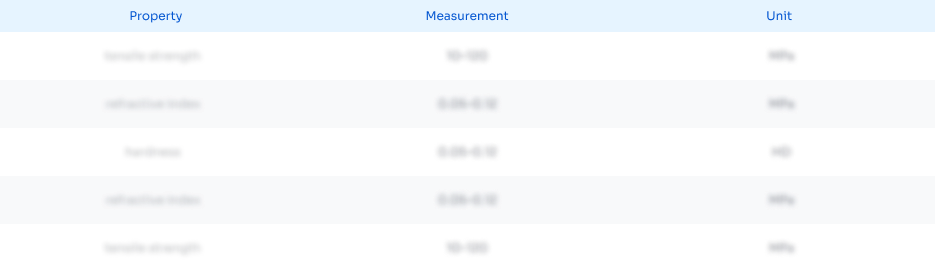
Abstract
Description
Claims
Application Information
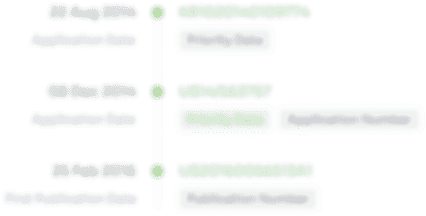
- R&D Engineer
- R&D Manager
- IP Professional
- Industry Leading Data Capabilities
- Powerful AI technology
- Patent DNA Extraction
Browse by: Latest US Patents, China's latest patents, Technical Efficacy Thesaurus, Application Domain, Technology Topic, Popular Technical Reports.
© 2024 PatSnap. All rights reserved.Legal|Privacy policy|Modern Slavery Act Transparency Statement|Sitemap|About US| Contact US: help@patsnap.com