Real-time cutter wear prediction method based on wavelet packet decomposition and deep learning
A wavelet packet decomposition, tool wear technology, applied in neural learning methods, character and pattern recognition, program control, etc., can solve problems such as difficult real-time prediction of tool wear
- Summary
- Abstract
- Description
- Claims
- Application Information
AI Technical Summary
Problems solved by technology
Method used
Image
Examples
Embodiment Construction
[0028] In order to make the object, technical solution and advantages of the present invention clearer, the present invention will be further described in detail below in conjunction with the accompanying drawings and embodiments. It should be understood that the specific embodiments described here are only used to explain the present invention, not to limit the present invention. In addition, the technical features involved in the various embodiments of the present invention described below can be combined with each other as long as they do not constitute a conflict with each other.
[0029] see figure 1 , figure 2 , image 3 and Figure 4 , the real-time prediction method of tool wear based on wavelet packet decomposition and deep learning provided by the present invention, the real-time prediction method mainly includes the following steps:
[0030] S1 data acquisition, storage and preprocessing. Specifically include the following sub-steps:
[0031] S11, install a t...
PUM
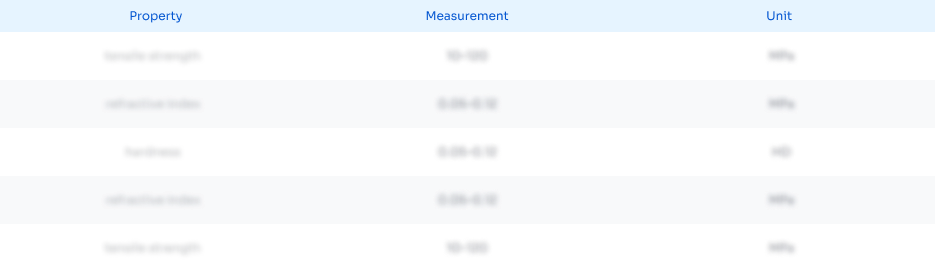
Abstract
Description
Claims
Application Information
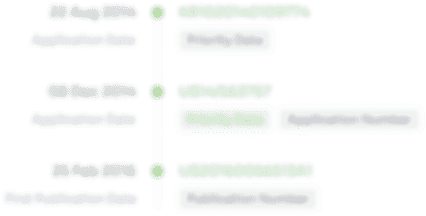
- R&D Engineer
- R&D Manager
- IP Professional
- Industry Leading Data Capabilities
- Powerful AI technology
- Patent DNA Extraction
Browse by: Latest US Patents, China's latest patents, Technical Efficacy Thesaurus, Application Domain, Technology Topic, Popular Technical Reports.
© 2024 PatSnap. All rights reserved.Legal|Privacy policy|Modern Slavery Act Transparency Statement|Sitemap|About US| Contact US: help@patsnap.com