Full convolution examination room target detection method based on cascade attention and point supervision mechanism
A technology of object detection and attention, applied in the cross-research field of educational science and image processing, can solve the problems of large difference in imaging size, blur, and intractability.
- Summary
- Abstract
- Description
- Claims
- Application Information
AI Technical Summary
Problems solved by technology
Method used
Image
Examples
Embodiment 1
[0036] Taking the special data set for examinee detection in the standardized test room as an example, the steps of the full convolution test target detection method based on the cascaded attention and point supervision mechanism are as follows (see figure 1 ):
[0037] (1) Build a full convolution test room target detection network model
[0038] Under the Pytorch framework, the feature extraction network output of the cascaded attention-based feature enhancement module is connected with the detection module using the point supervision branch to form a fully convolutional test room target detection network model based on the cascade attention and point supervision mechanism.
[0039] exist figure 2Among them, the full convolution test room target detection network model based on the cascaded attention and point supervision mechanism in this embodiment consists of a backbone network 1, a cascaded attention module 2, a feature pyramid network 3, and a full convolution detecti...
Embodiment 2
[0067] Taking the special data set for test taker detection in the standardized test room as an example, the steps of the full convolution test target detection method based on the cascaded attention and point supervision mechanism are as follows:
[0068] (1) Build a full convolution test room target detection network model
[0069] This step is the same as in Example 1.
[0070] (2) Training the full convolution test room target detection network model
[0071] (a) Setting the hyperparameters of the network
[0072] Take 700 images from the standardized examination room examinee detection data set as the training set, and 180 images as the test set. The pixel size of the training set input image is 1000×600, the data batch is 2, and the stochastic gradient descent method is used as the full convolution examination room. The optimizer of the target detection network model, the learning rate is initially set to 0.005 and decreased by 10 times at the 35th iteration, and the f...
Embodiment 3
[0077] Taking the special data set for test taker detection in the standardized test room as an example, the steps of the full convolution test target detection method based on the cascaded attention and point supervision mechanism are as follows:
[0078] (1) Build a full convolution test room target detection network model
[0079] This step is the same as in Example 1.
[0080] (2) Training the full convolution test room target detection network model
[0081] (a) Setting the hyperparameters of the network
[0082] Take 700 images from the standardized examination room examinee detection data set as the training set, and 180 images as the test set. The pixel size of the training set input image is 1000×600, the data batch is 2, and the stochastic gradient descent method is used as the full convolution examination room. The optimizer of the target detection network model, the learning rate is initially set to 0.015 and decreased by 10 times at the 40th iteration, and the f...
PUM
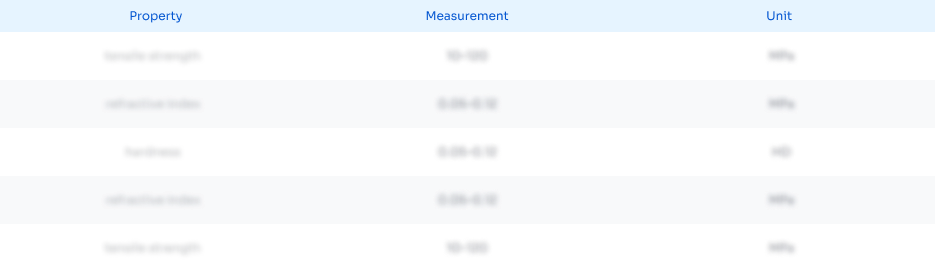
Abstract
Description
Claims
Application Information
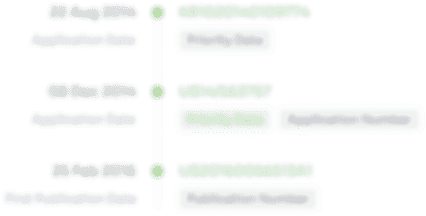
- R&D Engineer
- R&D Manager
- IP Professional
- Industry Leading Data Capabilities
- Powerful AI technology
- Patent DNA Extraction
Browse by: Latest US Patents, China's latest patents, Technical Efficacy Thesaurus, Application Domain, Technology Topic, Popular Technical Reports.
© 2024 PatSnap. All rights reserved.Legal|Privacy policy|Modern Slavery Act Transparency Statement|Sitemap|About US| Contact US: help@patsnap.com