Image classification method based on probability density distribution dictionary and Markov transfer features
A probability density distribution, dictionary technology, applied in character and pattern recognition, instruments, computer parts, etc., can solve the problems of large dimension of classification features, weak adaptability, and unsatisfactory robustness.
- Summary
- Abstract
- Description
- Claims
- Application Information
AI Technical Summary
Problems solved by technology
Method used
Image
Examples
Embodiment Construction
[0102] A kind of image classification method based on probability density distribution dictionary and Markov transition feature of the present invention, carry out according to the following steps:
[0103] Step 1. Input an image I with a size of B×B, and perform 3-layer discrete wavelet transform on it to obtain 9 high-frequency subbands: 3 horizontal subbands cH j ∈{cH 1 ,cH 2 ,cH 3}, 3 vertical subbands cV j ∈{cV 1 ,cV 2 ,cV 3} and 3 diagonal direction subbands cD j ∈{cD 1 ,cD 2 ,cD 3}, the j represents the scale of the high-frequency sub-band and j∈{1,2,3}, in this embodiment, let B=256;
[0104] Step 2. Count the normalization coefficient histograms of the 9 high-frequency subbands respectively;
[0105] Step 3. Set up a probability density distribution dictionary D with generalized Gaussian distribution, Cauchy distribution, Laplace distribution and α-stable distribution as distribution atoms;
[0106] Step 4. Fit the normalized coefficient histograms of the ...
PUM
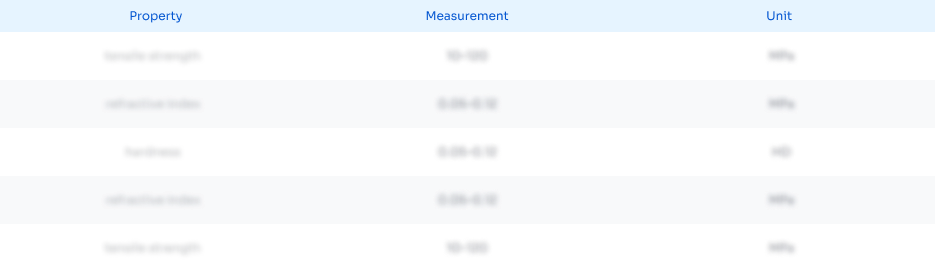
Abstract
Description
Claims
Application Information
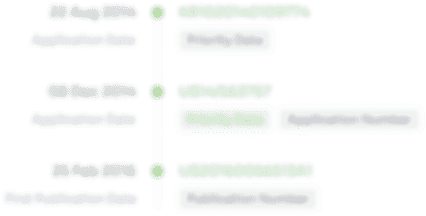
- R&D Engineer
- R&D Manager
- IP Professional
- Industry Leading Data Capabilities
- Powerful AI technology
- Patent DNA Extraction
Browse by: Latest US Patents, China's latest patents, Technical Efficacy Thesaurus, Application Domain, Technology Topic, Popular Technical Reports.
© 2024 PatSnap. All rights reserved.Legal|Privacy policy|Modern Slavery Act Transparency Statement|Sitemap|About US| Contact US: help@patsnap.com