Multi-source data deep fusion method based on deep learning
A technology of deep learning and multi-source data, applied in the field of deep fusion of multi-source data based on deep learning, can solve problems such as processing performance degradation and affecting data processing accuracy, and achieve the effect of improving tolerance
- Summary
- Abstract
- Description
- Claims
- Application Information
AI Technical Summary
Problems solved by technology
Method used
Image
Examples
Embodiment 1
[0040] Specifically, the embodiment of this application proposes a deep learning-based multi-source data deep fusion method, such as figure 1 shown, including:
[0041] 11. Obtain the relational data tables to be fused including the first data table and the second data table;
[0042] 12. Build a deep learning model, import training data into the deep learning model, perform word vectorization processing on the content in the fusion relational data table, and perform pattern matching on the processed data;
[0043] 13. Based on the similarity between the corresponding entities of the data, the data in the fusion relational data table is to be hierarchically sampled, and the sampled data is imported into the preset structural model for integration processing based on word vectors, and the trained data points are obtained. Bucket model, based on the data bucket model for entity-based data bucket processing;
[0044] 14. Determine whether the data in each bucket refers to the s...
PUM
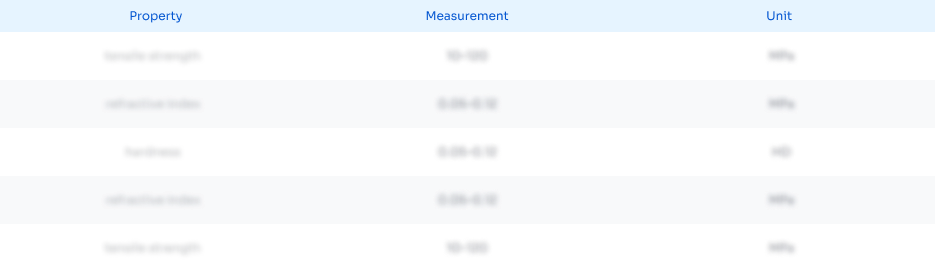
Abstract
Description
Claims
Application Information
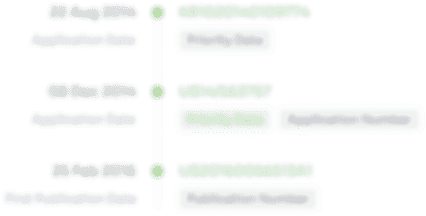
- R&D Engineer
- R&D Manager
- IP Professional
- Industry Leading Data Capabilities
- Powerful AI technology
- Patent DNA Extraction
Browse by: Latest US Patents, China's latest patents, Technical Efficacy Thesaurus, Application Domain, Technology Topic, Popular Technical Reports.
© 2024 PatSnap. All rights reserved.Legal|Privacy policy|Modern Slavery Act Transparency Statement|Sitemap|About US| Contact US: help@patsnap.com