Building energy consumption prediction method based on rough set and deep belief neural network
A deep neural network and building energy consumption technology, applied in neural learning methods, biological neural network models, predictions, etc., can solve problems such as low accuracy and difficulty in use, and solve problems such as insufficient practicability, insufficient accuracy, Improve the effectiveness of energy supply and demand management
- Summary
- Abstract
- Description
- Claims
- Application Information
AI Technical Summary
Problems solved by technology
Method used
Image
Examples
Embodiment 1
[0085] Surveyors conduct on-site detection of building energy consumption impact factor hourly data and power consumption hourly data for buildings such as civil public buildings, and collect one-to-one corresponding outdoor temperature, relative humidity, wind speed, outdoor solar irradiance, number of floors, Building area, building orientation, window-to-wall area ratio, external wall heat transfer coefficient, shading coefficient, building aspect ratio, roof heat transfer coefficient, lighting power density, personnel density, indoor temperature, per capita fresh air volume, chiller COP, air supply The hourly average value data of 20 building energy consumption factors such as temperature, fan efficiency and water pump efficiency and the hourly power consumption data representing building energy consumption form a 100 sets of raw data for rough compaction of building energy consumption factors For the sample set, see Table 4. The test period for each set of data is 1 hour....
PUM
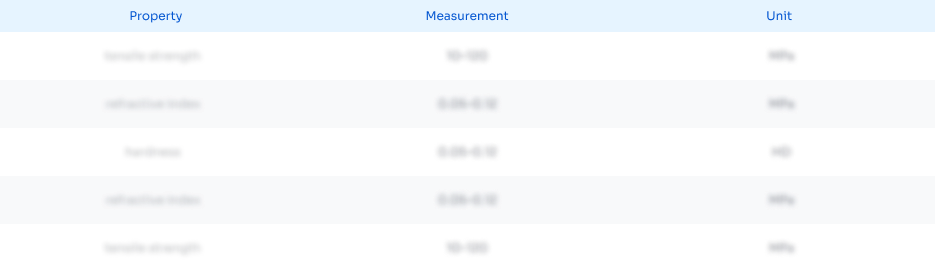
Abstract
Description
Claims
Application Information
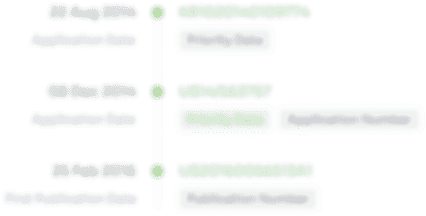
- R&D Engineer
- R&D Manager
- IP Professional
- Industry Leading Data Capabilities
- Powerful AI technology
- Patent DNA Extraction
Browse by: Latest US Patents, China's latest patents, Technical Efficacy Thesaurus, Application Domain, Technology Topic, Popular Technical Reports.
© 2024 PatSnap. All rights reserved.Legal|Privacy policy|Modern Slavery Act Transparency Statement|Sitemap|About US| Contact US: help@patsnap.com