Deep learning parallel computing architecture method and hyper-parameter automatic configuration optimization thereof
A parallel computing and deep learning technology, applied in the field of deep learning, can solve problems such as immature convergence, slow solution speed, unreasonable parameters, etc.
- Summary
- Abstract
- Description
- Claims
- Application Information
AI Technical Summary
Problems solved by technology
Method used
Image
Examples
Embodiment Construction
[0049] The principle of the present invention is to construct a parallel model framework for predicting spatio-temporal data based on the simple recurrent units (Simple Recurrent Units, SRU) of the recurrent neural network that realizes the parallel operation. This model architecture can accelerate the model in parallel to a certain extent, reduce the time required for model training and reasoning, and has little impact on the prediction performance of spatiotemporal data. At the same time, because the hyperparameters of the model deeply affect the performance of the model, such as the step size of time series data processing, the number of hidden layer units, the size of the convolution kernel and other hyperparameters, most of the current parameter tuning methods have their own drawbacks, so it is further proposed A method for automatic configuration of hyperparameters of the model based on parallel genetic algorithm is proposed. This method can automatically configure the h...
PUM
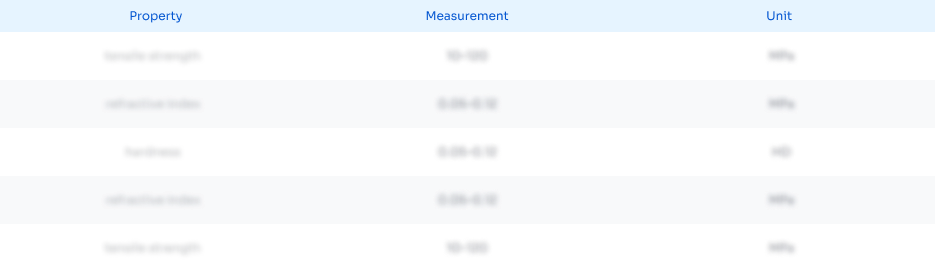
Abstract
Description
Claims
Application Information
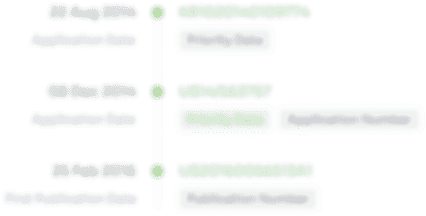
- R&D Engineer
- R&D Manager
- IP Professional
- Industry Leading Data Capabilities
- Powerful AI technology
- Patent DNA Extraction
Browse by: Latest US Patents, China's latest patents, Technical Efficacy Thesaurus, Application Domain, Technology Topic, Popular Technical Reports.
© 2024 PatSnap. All rights reserved.Legal|Privacy policy|Modern Slavery Act Transparency Statement|Sitemap|About US| Contact US: help@patsnap.com