Fine-grained action detection method of convolutional neural network based on multistage condition influence
A convolutional neural network and motion detection technology, applied in neural learning methods, biological neural network models, neural architectures, etc., to achieve excellent results, good universality and practicability, and improve reasoning ability and visual perception ability
- Summary
- Abstract
- Description
- Claims
- Application Information
AI Technical Summary
Problems solved by technology
Method used
Image
Examples
Embodiment Construction
[0041] The present invention proposes a fine-grained action detection method based on a convolutional neural network influenced by multi-level conditions, which fuses additional explicit knowledge in images with multi-level visual features. The proposed method is evaluated on the two most commonly used dataset benchmarks, namely HICO-DET and V-COCO. Experimental results show that the method of the present invention is superior to the existing methods.
[0042] Given an image I, use some off-the-shelf visual perception models to extract additional spatial semantic knowledge It is input to the MLCNet proposed by the present invention together with I In order to enhance the fine-grained action reasoning ability of CNN:
[0043]
[0044] where Ψ refers to the detected fine-grained action instance {(b h ,b o ,σ)}, where b h and b o are the bounding boxes of detected persons and objects, respectively, and σ belongs to the set of fine-grained action categories. The fine-g...
PUM
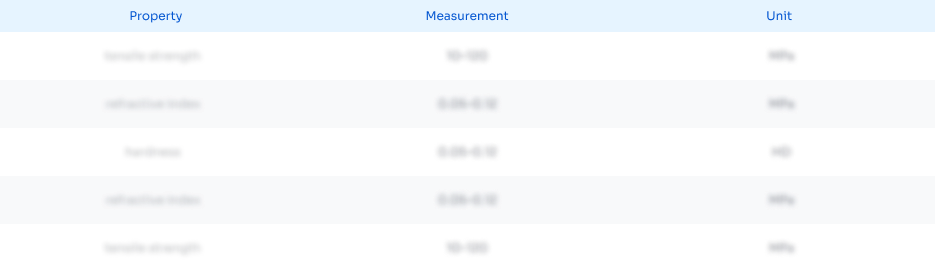
Abstract
Description
Claims
Application Information
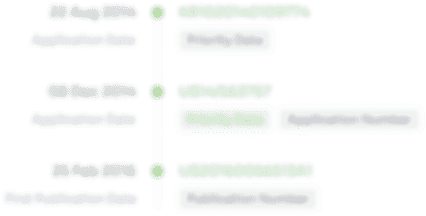
- R&D Engineer
- R&D Manager
- IP Professional
- Industry Leading Data Capabilities
- Powerful AI technology
- Patent DNA Extraction
Browse by: Latest US Patents, China's latest patents, Technical Efficacy Thesaurus, Application Domain, Technology Topic, Popular Technical Reports.
© 2024 PatSnap. All rights reserved.Legal|Privacy policy|Modern Slavery Act Transparency Statement|Sitemap|About US| Contact US: help@patsnap.com