Model-universal deep neural network representation visualization method and device
A convolutional neural network and representation technology, applied in the field of intelligence, which can solve problems such as weak interpretability, inability to trust model answers, and limiting the practical application of deep models.
- Summary
- Abstract
- Description
- Claims
- Application Information
AI Technical Summary
Problems solved by technology
Method used
Image
Examples
Embodiment 1
[0049] see figure 1 , a convolutional neural network representation visualization method, the method comprising:
[0050] Step S1: After the image to be visualized is input into the convolutional neural network, a first feature map is obtained, wherein the first feature map is the feature data generated by the layer to be visualized of the convolutional neural network;
[0051] Step S2: unpooling the first feature map to obtain a second feature map;
[0052] Step S3: correcting the second feature map by ReLU function to obtain a third feature map;
[0053] Step S4: Deconvolution or guided backpropagation of the third feature map to obtain the first visualized feature map;
[0054] Step S5: Displaying the first visualized feature map.
[0055] In the embodiment of the present disclosure, the feature activation of the layer to be visualized in the convolutional neural network model is displayed through anti-pooling, anti-activation, and deconvolution / guided backpropagation op...
Embodiment 2
[0078] see figure 2 , the convolutional neural network representation visualization device includes:
[0079] The first acquisition module 1 acquires a first feature map after the image to be visualized is input into the convolutional neural network, wherein the first feature map is the feature data generated by the layer to be visualized of the convolutional neural network;
[0080] Anti-pooling module 2, anti-pooling the first feature map to obtain a second feature map;
[0081] Correction module 3, correcting the second feature map through a ReLU function to obtain a third feature map;
[0082] Deconvolution module 4, deconvolution or guided backpropagation of the third feature map to obtain the first visualized feature map;
[0083] The first display module 5 is configured to display the first visualized feature map.
[0084] In one embodiment, the device also includes:
[0085] The second acquisition module acquires a fourth feature map, and the fourth feature map is...
Embodiment 3
[0096] Convolutional neural network training methods, including:
[0097] Execute the steps of any one of the convolutional neural network representation visualization methods described in Embodiment 1;
[0098] The verification result of the judgment of the convolutional neural network receiving the input;
[0099] If the verification result is correct, the image to be visualized is used as a training sample to train the convolutional neural network.
[0100] After outputting the first visualized feature map, the third visualized feature map or the third visualized feature map, the user can quickly judge the convolutional neural network based on the first visualized feature map, the third visualized feature map or the third visualized feature map Whether the judgment results are accurate, when the judgment basis and the judgment results are both accurate, the input verification result is correct, and when the verification result is correct, the image to be visualized is used...
PUM
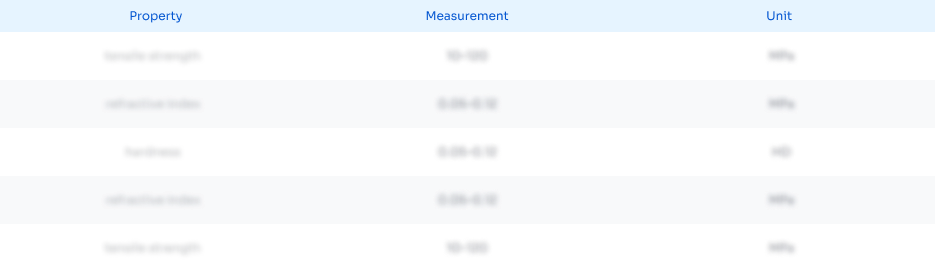
Abstract
Description
Claims
Application Information
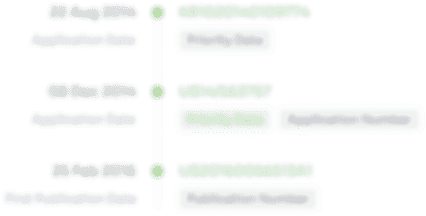
- R&D Engineer
- R&D Manager
- IP Professional
- Industry Leading Data Capabilities
- Powerful AI technology
- Patent DNA Extraction
Browse by: Latest US Patents, China's latest patents, Technical Efficacy Thesaurus, Application Domain, Technology Topic, Popular Technical Reports.
© 2024 PatSnap. All rights reserved.Legal|Privacy policy|Modern Slavery Act Transparency Statement|Sitemap|About US| Contact US: help@patsnap.com