Abnormal group detection method based on weighted dynamic network representation learning
A technology of dynamic network and detection method, applied in the direction of neural learning method, biological neural network model, instrument, etc., can solve the problems of not being able to learn the corresponding relationship between edges and weights well, and being unable to effectively identify abnormal weights, etc.
- Summary
- Abstract
- Description
- Claims
- Application Information
AI Technical Summary
Problems solved by technology
Method used
Image
Examples
Embodiment Construction
[0061] For a better understanding of the present invention, the meanings of some nouns appearing in the present invention are explained:
[0062] Weighted dynamic network: Weighted dynamic network is a weighted network that changes over time. A dynamic network containing n time slices is expressed as G={G 1 ,G 2 ,...,G t ,G t+1 ,...,G n}, where the tth time slice network G t =(V t ,E t ,W t ), V t is the set of vertices in the network, E t Represents the relationship between vertices for the set of edges, W t is the set of edge weights.
[0063] Weight exception: Given a dynamic network G={G 1 ,G 2 ,...,G t ,G t+1 ,...,G n}, for any time slice network G t =(V t ,E t ,W t ), for any edge e i ∈ E t , e i ={frm,to,w i}, where frm and to are the two endpoints of the edge, w t is the weight of the current edge, within the range of n time slices, the normal weight range of the edge with frm and to as endpoints is [w l ,w h ], if w i l or w i >w h then th...
PUM
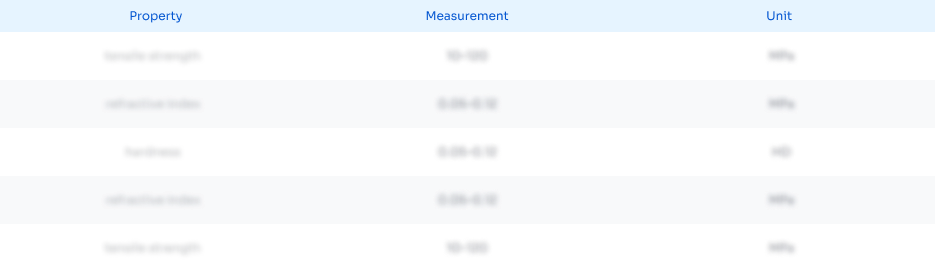
Abstract
Description
Claims
Application Information
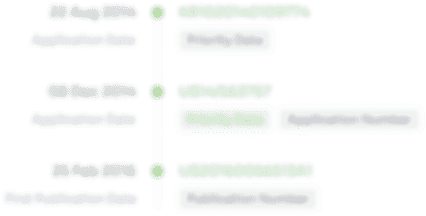
- Generate Ideas
- Intellectual Property
- Life Sciences
- Materials
- Tech Scout
- Unparalleled Data Quality
- Higher Quality Content
- 60% Fewer Hallucinations
Browse by: Latest US Patents, China's latest patents, Technical Efficacy Thesaurus, Application Domain, Technology Topic, Popular Technical Reports.
© 2025 PatSnap. All rights reserved.Legal|Privacy policy|Modern Slavery Act Transparency Statement|Sitemap|About US| Contact US: help@patsnap.com