Enteroscopy lymphoma auxiliary diagnosis system based on deep learning
An auxiliary diagnosis and deep learning technology, applied in image data processing, instrument, character and pattern recognition, etc., can solve problems such as high misdiagnosis rate, consumption of a lot of time, and delay in patient diagnosis time, so as to reduce workload and improve recognition. The effect of accuracy
- Summary
- Abstract
- Description
- Claims
- Application Information
AI Technical Summary
Problems solved by technology
Method used
Image
Examples
Embodiment 1
[0031] In one or more embodiments, a deep learning-based lymphoma auxiliary diagnosis system under enteroscopy is disclosed, including:
[0032] The image acquisition module is connected to the endoscope host through the acquisition card to obtain each frame of image information collected by the endoscope host;
[0033] The image preprocessing module is used to preprocess the collected image information; specifically includes:
[0034] Since the images are collected individually by the endoscope system in the clinic, it is necessary to remove the patient's private data in the images. In order to reduce the amount of calculation, it is necessary to remove the black frame and only keep the colored digestive tract area.
[0035] Through the black edge algorithm processing, zoom processing and normalization processing, each frame of the image is processed by the black edge algorithm to remove the redundant border of the endoscopic image and only retain the ROI area, and then use ...
Embodiment 2
[0049] In one or more embodiments, a terminal device is disclosed, which includes a processor and a computer-readable storage medium, the processor is used to implement instructions; the computer-readable storage medium is used to store multiple instructions, and the instructions are suitable for is loaded by the processor and executes the following processes:
[0050] Connect to the endoscope host through the acquisition card to obtain each frame of image information collected by the endoscope host;
[0051] Selecting a single frame of images with lymphoma lesions as a training sample, labeling the lesion area in the training sample, and generating labeling text information corresponding to the labeling position; the labeling area and the labeling text information corresponding to the area constitute a training set;
[0052] Construct an auxiliary diagnosis model, use the training set to optimize the training of the auxiliary diagnosis model; input the images to be detected i...
PUM
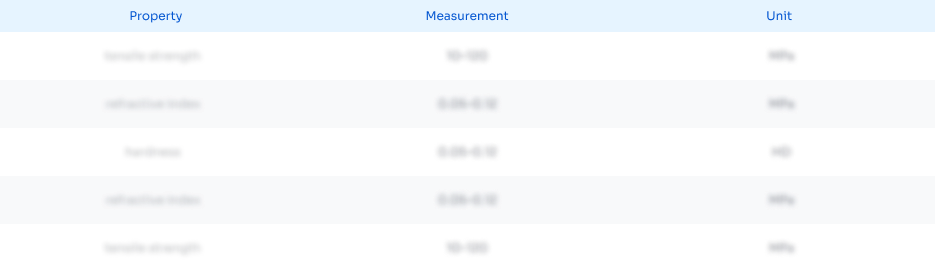
Abstract
Description
Claims
Application Information
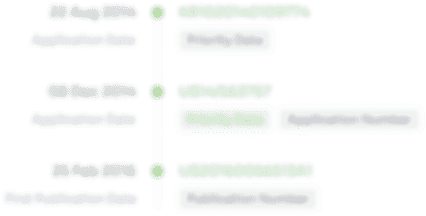
- R&D Engineer
- R&D Manager
- IP Professional
- Industry Leading Data Capabilities
- Powerful AI technology
- Patent DNA Extraction
Browse by: Latest US Patents, China's latest patents, Technical Efficacy Thesaurus, Application Domain, Technology Topic, Popular Technical Reports.
© 2024 PatSnap. All rights reserved.Legal|Privacy policy|Modern Slavery Act Transparency Statement|Sitemap|About US| Contact US: help@patsnap.com