Brain nuclear magnetic resonance abnormal image visualization method based on 2D CAM
A kind of nuclear magnetic resonance, abnormal image technology, applied in image enhancement, image analysis, image data processing and other directions, can solve the problem of invisible model learning and so on
- Summary
- Abstract
- Description
- Claims
- Application Information
AI Technical Summary
Problems solved by technology
Method used
Image
Examples
Embodiment Construction
[0056] The present invention will be further described in detail below in conjunction with the accompanying drawings and specific embodiments.
[0057] A method for visualization of brain MRI abnormal images based on 2D CAM, the specific method is as follows:
[0058] 1) Collect abnormal brain MRI images of patients as training samples;
[0059] 2) Use the training samples to train the 2D CAM, and determine the network parameters after training, namely the coefficient matrix W and the bias vector b value;
[0060] 21) Construct a 2D CAM model and initialize the network parameters randomly
[0061] Build 2D CAM models such as figure 1 As shown, the 2D CAM model includes an input layer, a convolution layer, a pooling layer, a global average pooling layer, a fully connected layer And the output layer (output layer), and initialize the 2DCAM model, that is, initialize the coefficient matrix W and the bias vector b value corresponding to all hidden layers and the output layer, s...
PUM
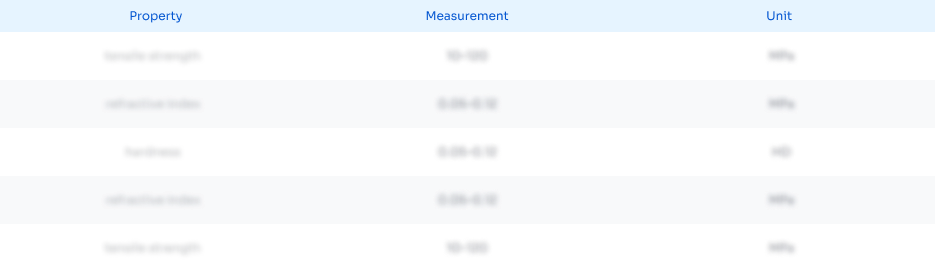
Abstract
Description
Claims
Application Information
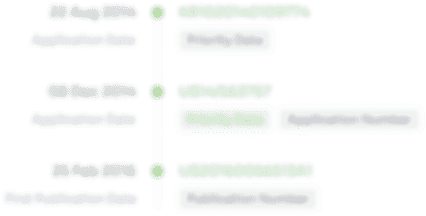
- R&D Engineer
- R&D Manager
- IP Professional
- Industry Leading Data Capabilities
- Powerful AI technology
- Patent DNA Extraction
Browse by: Latest US Patents, China's latest patents, Technical Efficacy Thesaurus, Application Domain, Technology Topic, Popular Technical Reports.
© 2024 PatSnap. All rights reserved.Legal|Privacy policy|Modern Slavery Act Transparency Statement|Sitemap|About US| Contact US: help@patsnap.com