A method for constructing depth image super-resolution reconstruction network based on color image guidance
A technology of super-resolution reconstruction and network construction, which is applied in the field of super-resolution reconstruction network construction based on color image guidance, can solve the problems of multiple texture areas and negative effects, and achieve high-quality and high-resolution effects
- Summary
- Abstract
- Description
- Claims
- Application Information
AI Technical Summary
Problems solved by technology
Method used
Image
Examples
Embodiment Construction
[0032] The following is attached figure 1 The present invention is described further, and the present invention comprises the following steps:
[0033] Step (1): Use the RGB-D camera to obtain the color image and depth image of the same scene
[0034] Using an RGB-D camera to get a low-resolution depth image I depth Its resolution is M*N, and a high-resolution color image I under the same viewing angle color Its resolution is rM*rN, where r is the magnification, and M and N are the height and width of the image respectively. For low-resolution depth images I depth The bicubic upsampling operation is performed to enlarge to rM*rN, and the initial low-quality high-resolution depth image is obtained and put the color image I color Convert to YCbCr color space, and take the Y channel image to get
[0035] Step (2): Construction of a dual-branch image feature extraction structure based on convolutional neural network. In the image feature extraction stage, the two branches...
PUM
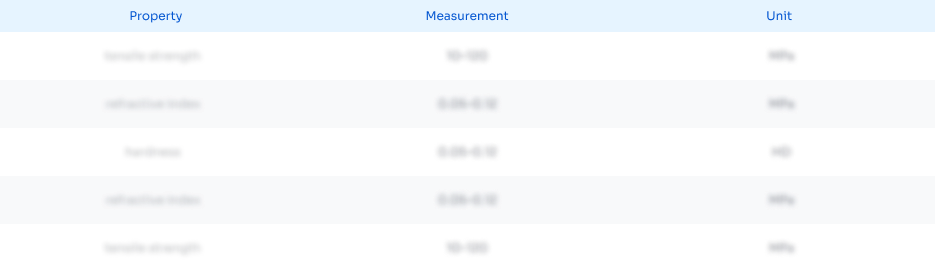
Abstract
Description
Claims
Application Information
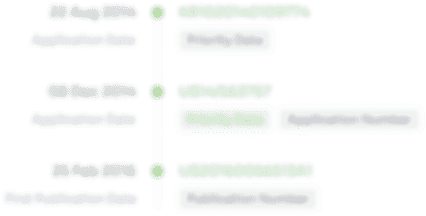
- R&D Engineer
- R&D Manager
- IP Professional
- Industry Leading Data Capabilities
- Powerful AI technology
- Patent DNA Extraction
Browse by: Latest US Patents, China's latest patents, Technical Efficacy Thesaurus, Application Domain, Technology Topic.
© 2024 PatSnap. All rights reserved.Legal|Privacy policy|Modern Slavery Act Transparency Statement|Sitemap