Deep learning-based sample generation method in industrial vision detection
A visual inspection and deep learning technology, applied in the field of sample generation based on deep learning, can solve the problems of long training time, high sample similarity, and few samples, etc., achieve low similarity, shorten training time, and avoid huge workload Effect
- Summary
- Abstract
- Description
- Claims
- Application Information
AI Technical Summary
Problems solved by technology
Method used
Image
Examples
Embodiment Construction
[0020] The present invention will now be described in further detail with reference to the accompanying drawings and preferred embodiments. These drawings are all simplified schematic diagrams, and only illustrate the basic structure of the present invention in a schematic manner, so they only show the structures related to the present invention.
[0021] like figure 1 Shown is a deep learning-based sample generation method in industrial visual inspection, including the following steps:
[0022] Firstly, the defects in the real defect pictures are extracted by conventional algorithms or manual annotation. The real defect pictures are the actual product pictures obtained on the defective product. Then, the extracted defects are randomly translated, rotated and projected by the P-picture algorithm. Paste it on the corresponding position of the non-defective sample picture, and do blurring, blurring and other processing around to achieve the fusion effect, thus producing a false...
PUM
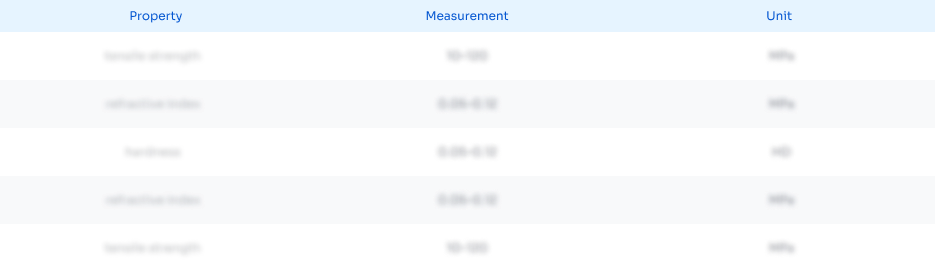
Abstract
Description
Claims
Application Information
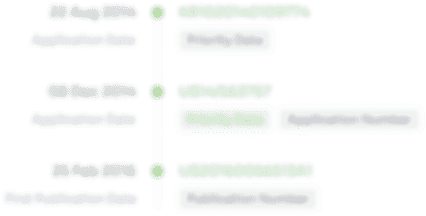
- R&D Engineer
- R&D Manager
- IP Professional
- Industry Leading Data Capabilities
- Powerful AI technology
- Patent DNA Extraction
Browse by: Latest US Patents, China's latest patents, Technical Efficacy Thesaurus, Application Domain, Technology Topic, Popular Technical Reports.
© 2024 PatSnap. All rights reserved.Legal|Privacy policy|Modern Slavery Act Transparency Statement|Sitemap|About US| Contact US: help@patsnap.com