A fast image denoising method based on octblock dense block
An image and fast technology, applied in the field of image processing, can solve the problems of increasing the difficulty of training, reducing network parameters, increasing the receptive field, etc., to avoid the increase of network complexity, reduce network parameters, and improve model efficiency.
- Summary
- Abstract
- Description
- Claims
- Application Information
AI Technical Summary
Problems solved by technology
Method used
Image
Examples
Embodiment Construction
[0054] The present invention will be further described in detail below with reference to examples and accompanying drawings, but the protection scope of the present invention is not limited thereto.
[0055] The present invention relates to a fast image denoising method based on OctBlock dense blocks, which comprises the following steps.
[0056] Step 1: Take the data set image as the image to be denoised; divide the image to be denoised into a training data set and a test data set.
[0057] In the present invention, 371 images are used as the training data set and the test data set. Segment 291 images as training dataset, of which 91 images are from Yang et al., and the other 200 images are from BerkeleySegmentation Dataset training set; the remaining images from 2 widely used benchmark datasets Set12 and BSD68, respectively As test datasets, among them, Set12 and BSD68 consist of natural scenes.
[0058] Step 2: Enhance and add noise to the training data set, and add noise...
PUM
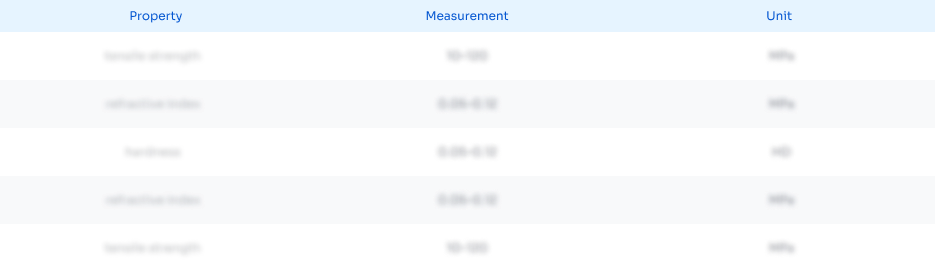
Abstract
Description
Claims
Application Information
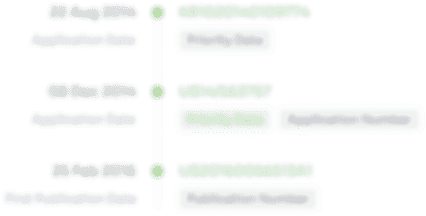
- R&D Engineer
- R&D Manager
- IP Professional
- Industry Leading Data Capabilities
- Powerful AI technology
- Patent DNA Extraction
Browse by: Latest US Patents, China's latest patents, Technical Efficacy Thesaurus, Application Domain, Technology Topic, Popular Technical Reports.
© 2024 PatSnap. All rights reserved.Legal|Privacy policy|Modern Slavery Act Transparency Statement|Sitemap|About US| Contact US: help@patsnap.com